Fault diagnosis method for automotive lithium battery based on multi-classification support vector machine algorithm
A technology of support vector machine and fault diagnosis, applied in neural learning methods, computing, data processing applications, etc., can solve problems such as the influence of fault diagnosis results, difficulty in data acquisition, and complex fault modes, so as to improve the speed of fault diagnosis and simplify diagnosis The process and steps are simple and reasonable
- Summary
- Abstract
- Description
- Claims
- Application Information
AI Technical Summary
Problems solved by technology
Method used
Image
Examples
Embodiment Construction
[0037] Support vector machine is an artificial intelligence algorithm for solving small-sample classification problems. This method is based on statistical learning theory and does not consider the characteristics of the battery itself and the internal reaction mechanism. It has unique solving advantages in small-sample statistics. There are local optimal solutions to the problem. The selection of support vector machine parameters will have a great impact on the results. If inappropriate parameters are selected, the accuracy of the model will be relatively poor. Therefore, to optimize the support vector machine parameters, cross-validation and grid search methods can be used. Using limited sample data, verify the fitness of the model for as many parameter combinations as possible.
[0038] Support vector machine is a binary classification algorithm. For fault diagnosis problems, an algorithm with multi-classification capability is required; multiple support vector machines can...
PUM
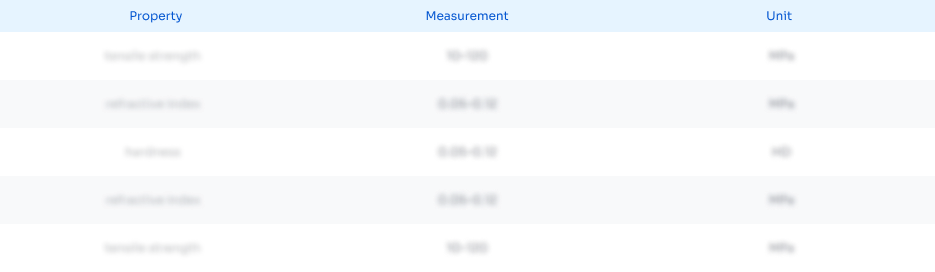
Abstract
Description
Claims
Application Information
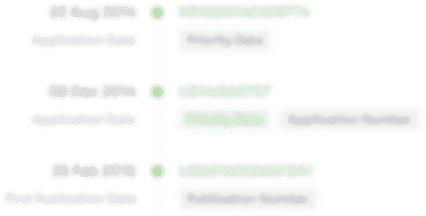
- R&D Engineer
- R&D Manager
- IP Professional
- Industry Leading Data Capabilities
- Powerful AI technology
- Patent DNA Extraction
Browse by: Latest US Patents, China's latest patents, Technical Efficacy Thesaurus, Application Domain, Technology Topic, Popular Technical Reports.
© 2024 PatSnap. All rights reserved.Legal|Privacy policy|Modern Slavery Act Transparency Statement|Sitemap|About US| Contact US: help@patsnap.com