Parameter identification of nonlinear system based on improved differential evolution algorithm
A nonlinear system and evolutionary algorithm technology, applied in the field of thermal automation, can solve problems such as algorithm stagnation, and achieve the effects of improved accuracy, easy understanding and implementation, and good adaptability
- Summary
- Abstract
- Description
- Claims
- Application Information
AI Technical Summary
Problems solved by technology
Method used
Image
Examples
Embodiment 1
[0062] In order to verify the effectiveness of the algorithm, several benchmark functions are used for testing. The test functions are Schwefel, Ackley, Rastrigin, Alpine, and Griewank functions, and the optimal solutions are all 0. The number of populations is set to be 10 times the dimension, and other parameters are set as follows: basic DE: F=0.6, CR=0.9, parameters of DEAFCR are: Fmax=1, Fmin=0.2, CRmax=1, CRmin=0.8. Table 1 shows the optimization results of these two algorithms for the 30-dimensional test function, each function is run 30 times, and the maximum number of iterations is 1000 times.
[0063] Table 1 Algorithm test results
[0064]
[0065] It can be seen from the test data in Table 1 that while maintaining the stability of the algorithm, the DEAFCR algorithm is smaller than the optimal solution and the average value of the DE algorithm. Therefore, the optimal solution and convergence accuracy searched by the DEAFCR algorithm are better than the basic DE...
Embodiment 2
[0067] In order to test the identification effect of the improved differential evolution algorithm in nonlinear fixed-length systems, this patent selects the transfer function model and the Hammerstein model for identification and simulation research. The results are compared with the genetic (GA) algorithm and the adaptive mutation rate and dynamic crossover mutation rate evolutionary difference algorithm (MCDE) to verify the effectiveness of the algorithm.
[0068] The transfer function model of the second-order inertia link plus pure time delay is shown in formula (8).
[0069]
[0070] The parameters to be identified are gain coefficient k, time constant T 1 , T 2 and delay time τ. The search range of parameters is 0≤k≤4,0≤T 1 ≤4,0≤T 2 ≤4,0≤τ≤2, the sampling period is 0.1s, the sampling data is 100, the input is random input u, the sampling time is 0≤t≤9.9, and the output signal y is calculated by the lsim function, y=lism(G, u,t).
[0071] The parameters in the s...
Embodiment 3
[0076] The Hammerstein model is composed of a memoryless nonlinear gain link and a linear subsystem in series. The model under the interference of colored noise can be described as:
[0077] A(q -1 )y(k)=B(q -1 )x(k)+C(q -1 )w(k) (9)
[0078] Considering the Hammerstein model in formula (9), the noise w(k) is Gaussian white noise with mean value 0 and variance 0.01. The input u(k) is Gaussian white noise with a mean of 0 and a variance of 0.2. Take: A(q -1 )=1-1.5q -1 +0.7q -2 , B(q -1 )=q -1 +0.5q -2 , C(q -1 )=1+1.5q -1 , x(k)=u(k)+0.5u 2 (k)+0.3u 3 (k)+0.1u 4 (k).
[0079] Therefore, the model has 8 parameters to be identified, a 1 、a 2 , b 1 , b 2 、c 1 、r 2 、r 3 、r 4 , the dimension is 8, the population size NP is 80, the number of iterations is 1000, the parameter search range is [-2,2], and the other parameters are set as above. The simulation results are shown in Table 3.
[0080] Table 3 Comparison of Hammerstein model parameter identification ...
PUM
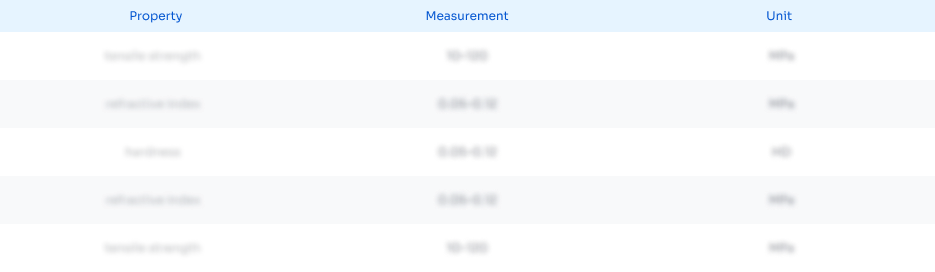
Abstract
Description
Claims
Application Information
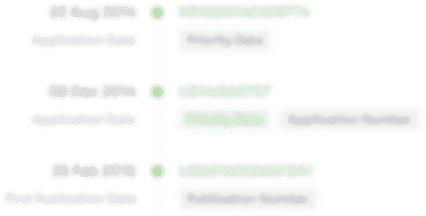
- R&D
- Intellectual Property
- Life Sciences
- Materials
- Tech Scout
- Unparalleled Data Quality
- Higher Quality Content
- 60% Fewer Hallucinations
Browse by: Latest US Patents, China's latest patents, Technical Efficacy Thesaurus, Application Domain, Technology Topic, Popular Technical Reports.
© 2025 PatSnap. All rights reserved.Legal|Privacy policy|Modern Slavery Act Transparency Statement|Sitemap|About US| Contact US: help@patsnap.com