A full convolutional neural network epilepsy focus segmentation method based on multi-mode images
A convolutional neural network, multi-modal technology, applied in the field of multi-modal image lesion segmentation, can solve problems such as damage to healthy brain tissue, reduce the effect of epilepsy surgical treatment, easy over-segmentation, etc., and achieve the effect of improving segmentation accuracy.
- Summary
- Abstract
- Description
- Claims
- Application Information
AI Technical Summary
Problems solved by technology
Method used
Image
Examples
Embodiment Construction
[0026] The specific implementation steps and effects of the present invention will be further described in detail below in conjunction with the accompanying drawings.
[0027] refer to figure 1 , the implementation steps of this example are as follows:
[0028] Step 1: Preprocessing the MRI / PET images.
[0029] 1a) Transform MRI images and PET images into the same resolution space, such as figure 2 shown, where figure 2 (a) is the MRI image before adjusting the resolution, figure 2 (b) is the PET image before adjusting the resolution, figure 2 (c) is the MRI image after adjusting the resolution, figure 2 (d) is the PET image after adjusting the resolution;
[0030] 1b) Match the MRI image with the PET image according to the image generation time and the outline of the skull to obtain a paired data set of brain multimodal images. Figure 4 (a), Figure 4 (b) MRI image and PET image after edge cropping, respectively;
[0031] 1c) Perform data expansion operations o...
PUM
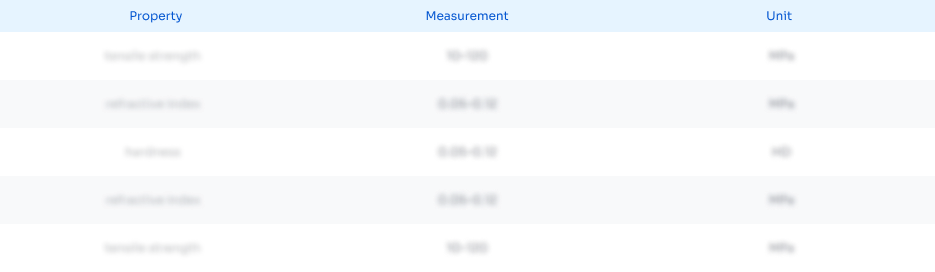
Abstract
Description
Claims
Application Information
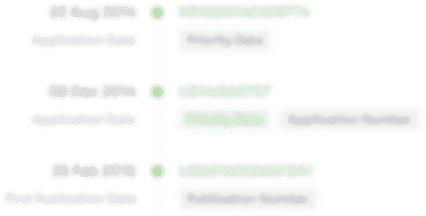
- R&D
- Intellectual Property
- Life Sciences
- Materials
- Tech Scout
- Unparalleled Data Quality
- Higher Quality Content
- 60% Fewer Hallucinations
Browse by: Latest US Patents, China's latest patents, Technical Efficacy Thesaurus, Application Domain, Technology Topic, Popular Technical Reports.
© 2025 PatSnap. All rights reserved.Legal|Privacy policy|Modern Slavery Act Transparency Statement|Sitemap|About US| Contact US: help@patsnap.com