Crowd Abnormal Event Detection Method Based on 3D Pyramid Image Generation Network
A technology of pyramid images and abnormal events, applied in the field of graphic recognition, can solve problems such as many steps, unfavorable distinction between normal images and abnormal images, and poor shadow suppression effect
- Summary
- Abstract
- Description
- Claims
- Application Information
AI Technical Summary
Problems solved by technology
Method used
Image
Examples
Embodiment
[0104] The method for detecting abnormal crowd events based on the three-dimensional pyramid image generation network of the present embodiment, the specific steps are as follows:
[0105] In the first step, the video of crowd activities is converted into a sequence of images:
[0106] Get a group of video sequences of crowd activities, use OpenCV to extract N frames of images f from any crowd video i i1 ,f i2 ,..., f iN The sequence of images that make up video i, denoted as F i {f i1 ,f i2 ,..., f id ,..., f iN}, where f id Represents the dth frame image of the image sequence extracted from video i, N is 200, for the obtained image sequence F i The images in the middle are standardized, and the size of the images is normalized to M×M pixels, and M is 256; the set of image sequences extracted from all video sequences is T{F 1 , F 2 ,...,F i ,...,F q}, where q represents the number of video sequences, F i Represents the image sequence of the i-th video, denoted as...
PUM
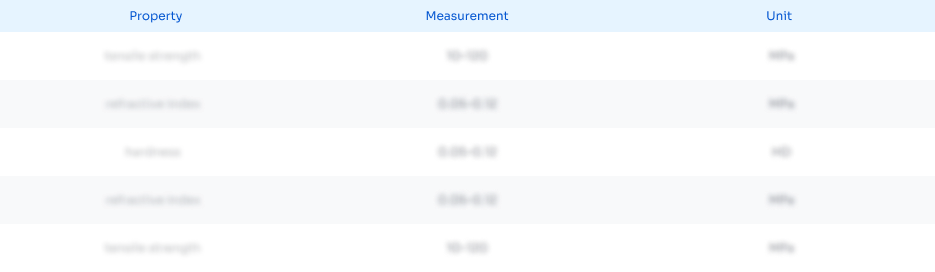
Abstract
Description
Claims
Application Information
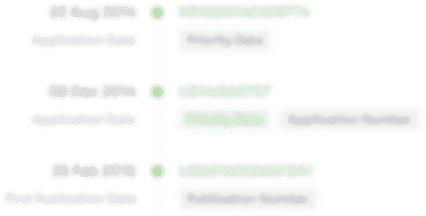
- R&D Engineer
- R&D Manager
- IP Professional
- Industry Leading Data Capabilities
- Powerful AI technology
- Patent DNA Extraction
Browse by: Latest US Patents, China's latest patents, Technical Efficacy Thesaurus, Application Domain, Technology Topic, Popular Technical Reports.
© 2024 PatSnap. All rights reserved.Legal|Privacy policy|Modern Slavery Act Transparency Statement|Sitemap|About US| Contact US: help@patsnap.com