Method for carrying out tree species classification on hyperspectral data of artificial forest by using three-dimensional convolutional neural network
A three-dimensional convolution and neural network technology, applied in neural learning methods, biological neural network models, neural architectures, etc., can solve problems such as low precision and low efficiency, and achieve high precision, accurate classification results, and high classification accuracy.
- Summary
- Abstract
- Description
- Claims
- Application Information
AI Technical Summary
Problems solved by technology
Method used
Image
Examples
Embodiment Construction
[0016] The present invention: a method of using a three-dimensional convolutional neural network to classify plantation forest hyperspectral data, said method comprising the following steps:
[0017] Step 1: Perform routine preprocessing on the original hyperspectral image data, including radiometric correction, geometric correction, atmospheric correction, and terrain correction.
[0018] Step 2: Construct the sample data, with the target pixel as the center, a space-spectral cube with a size of 11×11×B and the corresponding label l as the sample data, where B represents the number of bands of the image, and stratified sampling is used on this basis According to the method, the constructed labeled cube samples are divided into training data set, verification data set and test data set according to a certain proportion.
[0019] Step 3: The construction of the three-dimensional convolutional neural network. First, the cube data with a dimension of 11×11×B obtained in step 2 is...
PUM
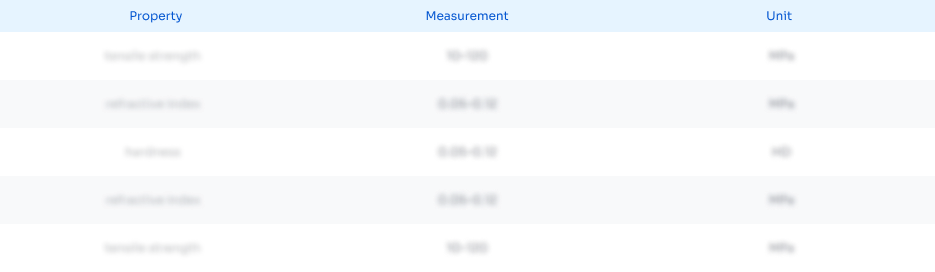
Abstract
Description
Claims
Application Information
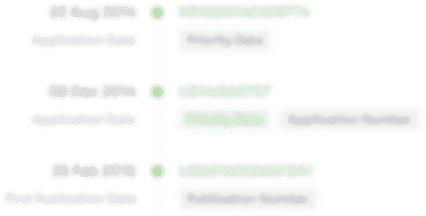
- R&D Engineer
- R&D Manager
- IP Professional
- Industry Leading Data Capabilities
- Powerful AI technology
- Patent DNA Extraction
Browse by: Latest US Patents, China's latest patents, Technical Efficacy Thesaurus, Application Domain, Technology Topic.
© 2024 PatSnap. All rights reserved.Legal|Privacy policy|Modern Slavery Act Transparency Statement|Sitemap