Mechanical equipment residual service life prediction method and system
A technology for life prediction and mechanical equipment, applied in neural learning methods, computer-aided design, design optimization/simulation, etc., can solve problems that restrict wide application, difficulty in obtaining physical degradation models, and difficulty in migration
- Summary
- Abstract
- Description
- Claims
- Application Information
AI Technical Summary
Problems solved by technology
Method used
Image
Examples
Embodiment 1
[0039] When performing maintenance work on mechanical equipment, if the remaining service life of the equipment can be accurately predicted, the life warning value of the equipment can be known in advance, and the operator will stop the equipment for inspection based on the warning value, and eliminate potential failure factors of the equipment. Operations such as equipment maintenance, replacement of parts, replacement of spare equipment, etc., avoid equipment failure during operation, and predict and "cure the disease" before the "disease" of the equipment. In this way, accidents caused by equipment failure can be avoided, economic losses can be reduced, and personnel safety can be protected; maintenance plans can be formulated in advance according to the life prediction results, and spare parts or spare equipment can be purchased in advance to reduce downtime, improve maintenance efficiency, and reduce transportation costs. maintenance cost; according to the forecast results...
Embodiment 2
[0074] Such as Figure 9 As shown, this embodiment provides a system for predicting the remaining service life of mechanical equipment, including: a historical database, a life prediction model and a full life cycle database;
[0075] The historical database is to store all the state monitoring data accumulated by the equipment of the same model and under the same working conditions, and use it as training data to train the life prediction model;
[0076]The life prediction model includes constructing a deep neural network life prediction model, the deep neural network life prediction model uses a time convolution network as a feature extraction algorithm, and a long short-term memory network is constructed as a regression prediction algorithm;
[0077] The life cycle database stores the collected real-time operation data of the tested equipment, and constructs the collected real-time running data of the tested equipment into a life prediction data set with time series charact...
PUM
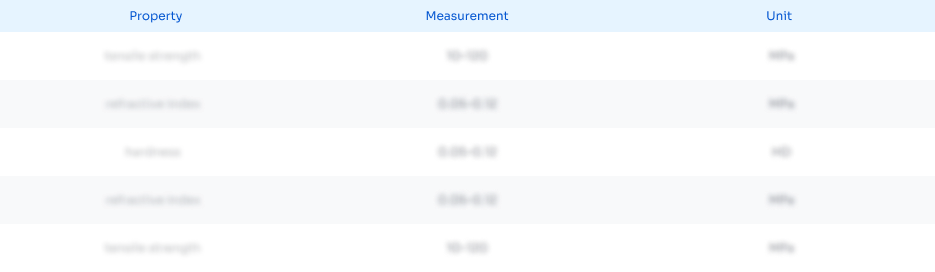
Abstract
Description
Claims
Application Information
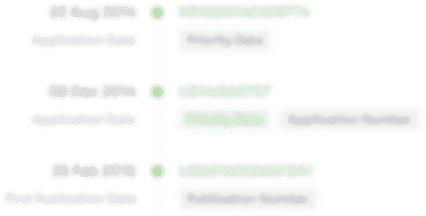
- R&D Engineer
- R&D Manager
- IP Professional
- Industry Leading Data Capabilities
- Powerful AI technology
- Patent DNA Extraction
Browse by: Latest US Patents, China's latest patents, Technical Efficacy Thesaurus, Application Domain, Technology Topic, Popular Technical Reports.
© 2024 PatSnap. All rights reserved.Legal|Privacy policy|Modern Slavery Act Transparency Statement|Sitemap|About US| Contact US: help@patsnap.com