Optimal feature vector design method for carrying out reverse photolysis based on machine learning
A technology of machine learning and optimal features, applied in machine learning, neural learning methods, computer-aided design, etc., can solve the problems of complex feature vector extraction layer, lack of physical meaning, and difficult training, so as to shorten training time and simplify neural networks. network effect
- Summary
- Abstract
- Description
- Claims
- Application Information
AI Technical Summary
Problems solved by technology
Method used
Image
Examples
Embodiment Construction
[0040] Attached below Figure 1-15 , the specific embodiment of the present invention will be further described in detail.
[0041]It should be noted that the optimal eigenvector design method for the reverse lithography solution based on machine learning in the present invention is used to predict the value of the reverse lithography solution. Among them, the value of the reverse lithography solution can be applied to reverse lithography based on machine learning, optical proximity correction (OPC) based on machine learning, hotspot detection in lithography based on machine learning, etc. The optimal eigenvector design method can be used for Computational lithography (inverse lithography, optical proximity correction, lithographic hotspot detection) for immersion lithography can also be used for computational lithography (inverse lithography, optical proximity correction, lithographic hotspot detection) for EUV lithography.
[0042] see figure 1 , figure 1 Shown is an idea...
PUM
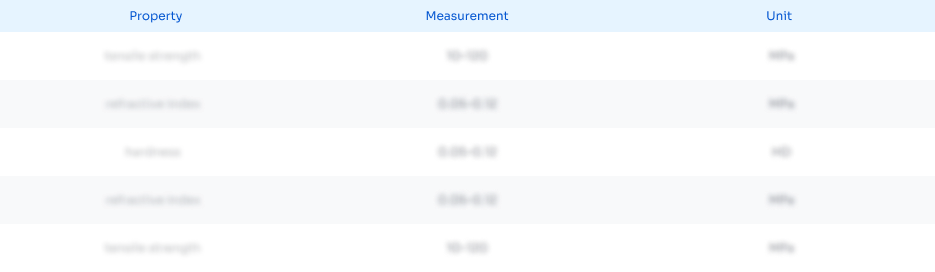
Abstract
Description
Claims
Application Information
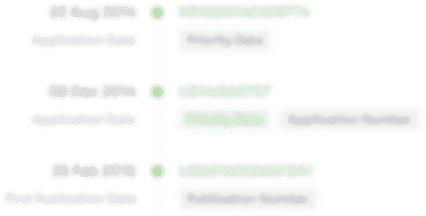
- R&D Engineer
- R&D Manager
- IP Professional
- Industry Leading Data Capabilities
- Powerful AI technology
- Patent DNA Extraction
Browse by: Latest US Patents, China's latest patents, Technical Efficacy Thesaurus, Application Domain, Technology Topic, Popular Technical Reports.
© 2024 PatSnap. All rights reserved.Legal|Privacy policy|Modern Slavery Act Transparency Statement|Sitemap|About US| Contact US: help@patsnap.com