Multi-working-condition power system performance prediction method and system based on Gaussian process regression
A Gaussian process regression, dynamic system technology, applied in information technology support systems, registration/instruction vehicle operation, complex mathematical operations, etc., can solve problems such as poor generalization ability, and achieve the effect of reducing experiment cost and difficulty
- Summary
- Abstract
- Description
- Claims
- Application Information
AI Technical Summary
Problems solved by technology
Method used
Image
Examples
Embodiment 1
[0089] The multi-working-condition power system performance prediction system based on Gaussian process regression disclosed in this embodiment is used for pure oil-powered power equipment manufacturers to predict the harmful gas emissions of the equipment under multiple working conditions before leaving the factory. A detailed description is given below.
[0090] Conventional working condition data acquisition unit: As an example and not limitation, the data acquisition unit can be a temperature sensor, speed sensor, torque sensor, pressure sensor, automobile exhaust detector, smoke sensor, etc. installed directly on the power system equipment as a sensing device , used to collect the input of the power system (required speed, required torque, gear position, driving mode, a total of 4 items), output (a certain harmful gas such as CO, NO X etc.) and environment (the temperature of the vehicle power system, atmospheric pressure, and the quantified usage of the vehicle's non-pow...
Embodiment 2
[0105] This embodiment is improved on the basis of Embodiment 1, and the main effect of the improvement is to increase the computing speed of the server unit.
[0106] In the sequential sampling unit of embodiment 1, such as image 3 As shown, every time a cycle is performed, the method of minimizing the negative logarithmic marginal likelihood function must be re-used to determine the hyperparameters of the Gaussian process regression model, and the particle swarm optimization algorithm used to solve the optimization problem has a slow convergence speed. This results in slower calculations for the entire server unit. On the other hand, the function Q in the sequential sampling algorithm in Embodiment 1 uses the L1 norm to represent the "gap" of the probability density distribution between the upper and lower confidence intervals. Since the L1 norm is more suitable for local sampling, using the L1 norm at the beginning will also result in a slower calculation speed for the en...
PUM
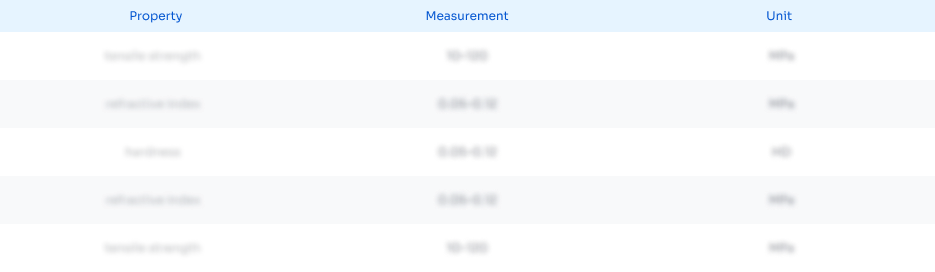
Abstract
Description
Claims
Application Information
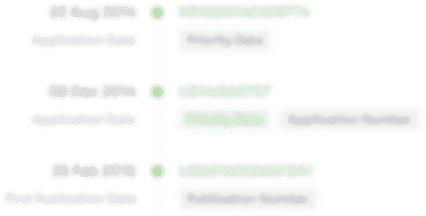
- R&D Engineer
- R&D Manager
- IP Professional
- Industry Leading Data Capabilities
- Powerful AI technology
- Patent DNA Extraction
Browse by: Latest US Patents, China's latest patents, Technical Efficacy Thesaurus, Application Domain, Technology Topic.
© 2024 PatSnap. All rights reserved.Legal|Privacy policy|Modern Slavery Act Transparency Statement|Sitemap