Weather image intelligent identification method and system based on multi-depth convolutional neural network fusion
A convolutional neural network and weather image technology, applied in the field of weather image intelligent recognition methods and systems, can solve the problems of ineffective guarantee of deep learning network training data quality and inefficient image features, etc.
- Summary
- Abstract
- Description
- Claims
- Application Information
AI Technical Summary
Problems solved by technology
Method used
Image
Examples
Embodiment 1
[0068] The overall implementation process of a weather image intelligent recognition method based on multi-depth convolutional neural network fusion is as follows: figure 1 As shown, proceed as follows:
[0069]Step A: Collect images of 9 weather phenomena including rime, rime, rain, snow, hail, dew, frost, fog or haze, and icing, and perform data cleaning, data amplification, and standardization processing on weather images, and formulate them as For a data set in a certain format, the overall steps are as follows figure 2 shown.
[0070] 1) Data Analysis
[0071] According to the collected 9,000 images of nine different weather conditions, the data set is constructed, and the image labels are 1-9, corresponding to rime, rime, rain, snow, hail, dew, frost, fog or haze, and icing. All the data is divided into training set and test set according to the ratio of 2:1.
[0072] Analyzing the data, the distribution of the number of images with different labels in the training ...
Embodiment 2
[0111] Automatic recognition of individual weather images. The first step is the construction of the training data set, collecting 6,000 images of 9 weather phenomena related to rime, rime, rain, snow, hail, dew, frost, fog or haze, and icing. First, analyze the data and perform data cleaning. Class balancing, data augmentation, and normalization to a dataset of size 512×512.
[0112]In the second step, four deep convolutional neural network models are selected: ResNe152, DenseNet169, ResNext101_64x4d, SE_ResNeXt101_32x4d, modify the fully connected layer of the network, and add a 27×1 fully connected layer before the output layer to form the feature layer of the network. The loss function of the network is shown in formula (1), and its weight parameters are set according to Table 1. The built network needs to be trained first. It is recommended to use GPU to accelerate the training process. The training batch size is 32. The optimizer is Adam and the initial learning rate is...
PUM
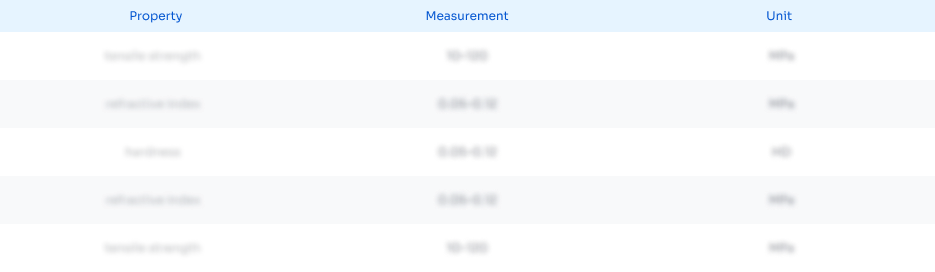
Abstract
Description
Claims
Application Information
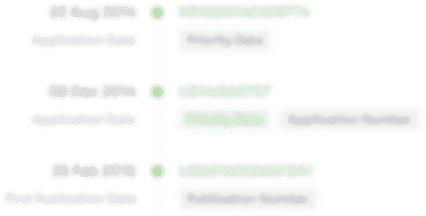
- R&D Engineer
- R&D Manager
- IP Professional
- Industry Leading Data Capabilities
- Powerful AI technology
- Patent DNA Extraction
Browse by: Latest US Patents, China's latest patents, Technical Efficacy Thesaurus, Application Domain, Technology Topic.
© 2024 PatSnap. All rights reserved.Legal|Privacy policy|Modern Slavery Act Transparency Statement|Sitemap