Space spectrum full convolution hyperspectral image classification method based on superpixel sample expansion
A hyperspectral image and sample expansion technology, applied in neural learning methods, instruments, biological neural network models, etc., can solve the problem of high cost of hyperspectral image labeling, achieve stable and reliable classification results, avoid highly redundant training data, Enhanced Robustness Effects
- Summary
- Abstract
- Description
- Claims
- Application Information
AI Technical Summary
Problems solved by technology
Method used
Image
Examples
Embodiment Construction
[0055] The invention provides a space-spectrum full-convolution hyperspectral image classification method based on superpixel sample expansion, input hyperspectral images; obtain training sets and test sets; perform principal component analysis and dimensionality reduction on hyperspectral images; dimensionality reduction As a result, perform entropy superpixel segmentation; generate pseudo-label samples; update the training set; perform data preprocessing on hyperspectral images; input fully convolutional neural networks; train fully convolutional neural networks to classify hyperspectral images; repeat the above operations And vote; output hyperspectral classification results. The present invention uses entropy rate superpixel segmentation results to expand pseudo-label samples, fully utilizes the spatial prior information of hyperspectral images, increases the number of samples, alleviates the problem of network over-fitting, and effectively improves high-resolution images u...
PUM
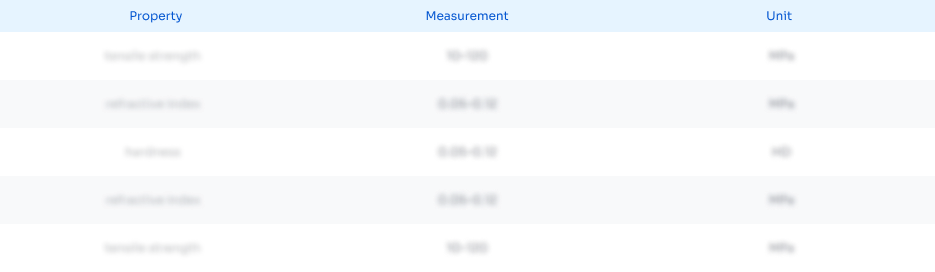
Abstract
Description
Claims
Application Information
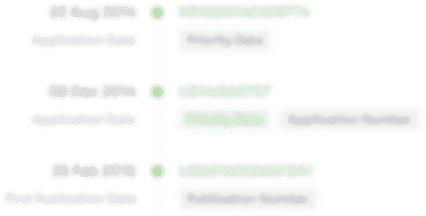
- R&D
- Intellectual Property
- Life Sciences
- Materials
- Tech Scout
- Unparalleled Data Quality
- Higher Quality Content
- 60% Fewer Hallucinations
Browse by: Latest US Patents, China's latest patents, Technical Efficacy Thesaurus, Application Domain, Technology Topic, Popular Technical Reports.
© 2025 PatSnap. All rights reserved.Legal|Privacy policy|Modern Slavery Act Transparency Statement|Sitemap|About US| Contact US: help@patsnap.com