Multi-modal data knowledge information extraction method based on deep-width joint neural network
A technology that combines neural and information extraction, applied in biological neural network models, neural learning methods, digital data information retrieval, etc. Achieve the effect of improving robustness, low dimensionality, and strong robustness
- Summary
- Abstract
- Description
- Claims
- Application Information
AI Technical Summary
Problems solved by technology
Method used
Image
Examples
Embodiment Construction
[0057] The present invention will be further described below in conjunction with specific examples.
[0058] Such as figure 1 As shown, the multimodal data knowledge information extraction method based on the deep-width joint neural network provided by this embodiment includes the following steps:
[0059] 1) Collect the multi-modal data logs generated by the intelligent manufacturing factory system in the daily assembly line, including voice, text, image and other types of multi-modal data, and preprocess the data, add the log samples to Kafka as the In the distributed log system implemented on the basis, since a large number of samples are processed, the processed data samples are stored in the storage module of the Hadoop distributed file system;
[0060] Preprocessing of data logs produced by smart manufacturing factories mainly includes the following operations: Data noise filtering and processing of missing values of data features. The processing of missing values o...
PUM
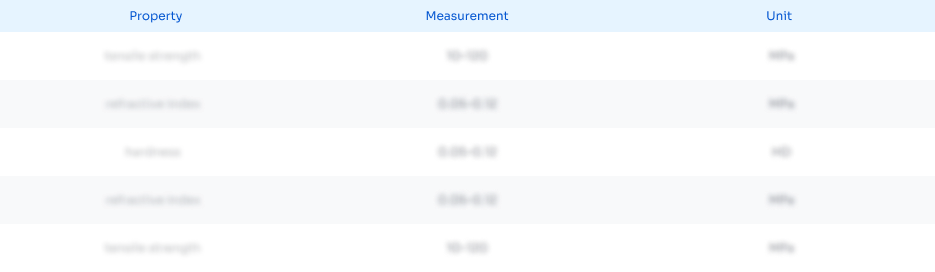
Abstract
Description
Claims
Application Information
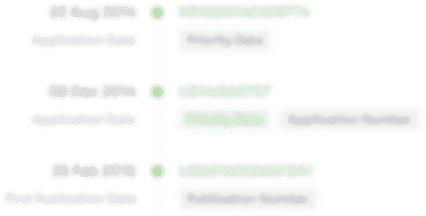
- R&D Engineer
- R&D Manager
- IP Professional
- Industry Leading Data Capabilities
- Powerful AI technology
- Patent DNA Extraction
Browse by: Latest US Patents, China's latest patents, Technical Efficacy Thesaurus, Application Domain, Technology Topic.
© 2024 PatSnap. All rights reserved.Legal|Privacy policy|Modern Slavery Act Transparency Statement|Sitemap