Signal-dependent noise parameter estimation method based on improved density peak clustering
A technology of noise parameters and density peaks, applied in computing, computer components, instruments, etc., can solve problems such as ignoring cluster centers, affecting clustering accuracy, and ignoring clustering in sparse areas, so as to improve estimation accuracy and benefit images. Effects of denoising and improving accuracy
- Summary
- Abstract
- Description
- Claims
- Application Information
AI Technical Summary
Problems solved by technology
Method used
Image
Examples
Embodiment Construction
[0037] Below in conjunction with the present invention is further explained; The original image used in the present embodiment, originates from the image that CMOS image sensor collects, and the noise in the original image is that in the acquisition process, the denoising module of CMOS image sensor produces depends on the signal noise component.
[0038] A signal-dependent noise parameter estimation method based on improved density peak clustering, which specifically includes the following steps:
[0039] Step 1. Using a sliding window with a size of 16*16, in the order from top to bottom and from left to right, slide the distance of one pixel each time, and extract n pieces of size 16*16 from the original image containing noise. of samples. Calculate the mean mean, entropy and gradient grad for each sample as the characteristic data of the sample:
[0040]
[0041]
[0042]
[0043]Among them, gray represents the size of the gray value, and p(gray) represents the ...
PUM
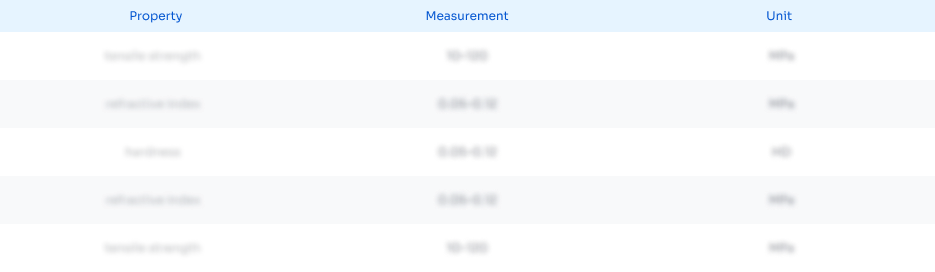
Abstract
Description
Claims
Application Information
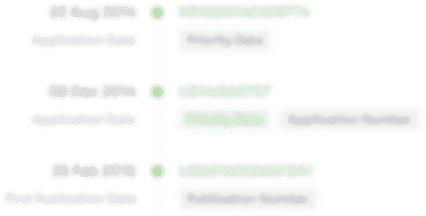
- R&D Engineer
- R&D Manager
- IP Professional
- Industry Leading Data Capabilities
- Powerful AI technology
- Patent DNA Extraction
Browse by: Latest US Patents, China's latest patents, Technical Efficacy Thesaurus, Application Domain, Technology Topic.
© 2024 PatSnap. All rights reserved.Legal|Privacy policy|Modern Slavery Act Transparency Statement|Sitemap