Intelligent antenatal fetal heart monitoring model
A model and intelligent technology, applied in the measurement of pulse rate/heart rate, medical science, sensors, etc., can solve the problems of high cost, low specificity, increased stillbirth rate, etc., and achieve improved classification performance, excellent denoising ability, and classification performance Enhanced effect
- Summary
- Abstract
- Description
- Claims
- Application Information
AI Technical Summary
Problems solved by technology
Method used
Image
Examples
Embodiment 1
[0039] The present invention provides an intelligent prenatal fetal heart rate monitoring model based on a multimodal feature deep learning strategy. The model includes the following steps:
[0040] S1: Obtain the original CTG signal data including fetal heart rate signal and uterine contraction signal and the basic information of pregnant woman including pregnant woman's age and gestational week;
[0041] S2: Preprocessing the above-mentioned fetal heart rate signal, uterine contraction signal, pregnant woman's age and gestational week to form a multimodal feature case set;
[0042] The preprocessing includes interpolating or deleting the fetal heart rate signal and the uterine contraction signal, and interpolating the age of the pregnant woman.
[0043] The preprocessing also includes segmenting the interpolated or deleted fetal heart rate signal before standardizing the interpolated or deleted fetal heart rate signal to obtain a fetal heart rate signal with a signal length ...
Embodiment 2
[0094] In order to verify the influence of different convolutional neural network structures on the classification performance of the intelligent prenatal fetal heart rate monitoring model of the present invention, the present invention designs eight convolutional neural networks with different structures, and conducts comparative analysis with verification example 2. The experimental results are shown in Table 2.
[0095] Eight different convolutional neural networks are, 6C2D: 6-layer convolutional layer, 2-layer fully connected layer (the same below); 6C3D; 6C4D; 6C6D; 3C5D; 4C5D; 5C5D; 7C5D. The network structure of Verification Example 1 is 6C5D, that is, 6 convolutional layers and 5 fully connected layers. The last neuron of all networks is 2, and the activation function is a fully connected layer of sigmoid to output the probability distribution.
[0096] Table 2 Comparative analysis results of classification performance of convolutional neural networks with different ...
Embodiment 3
[0100] In order to compare the classification effect of this experimental model, the present invention uses KNN, SVM, DT, RF, BP, Bayes, GBDT machine learning models based on clinical features as the model comparison of this experiment. The experimental results are shown in Table 3.
[0101] The k-algorithm of the nearest neighbor (k-Nearest Neighbor, KNN) is a relatively mature method in theory. The idea is that, in a particular space, a sample also belongs to that class, although most of the k samples closest to it belong to that class.
[0102] A vector machine (Support Vector Machine, SVM) is an advanced linear classifier that classifies data according to a supervised learning method, and the maximum resolution is the maximum approximate mid-region distance of the learning samples.
[0103] Backpropagation (Back-propagation, BP) neural network is a multi-layer feed-forward network formed by using the error inversion algorithm. BP can study and store a large number of inp...
PUM
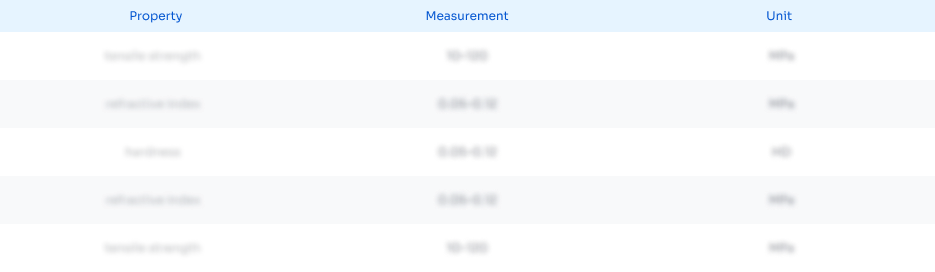
Abstract
Description
Claims
Application Information
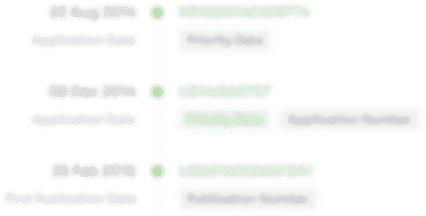
- R&D Engineer
- R&D Manager
- IP Professional
- Industry Leading Data Capabilities
- Powerful AI technology
- Patent DNA Extraction
Browse by: Latest US Patents, China's latest patents, Technical Efficacy Thesaurus, Application Domain, Technology Topic.
© 2024 PatSnap. All rights reserved.Legal|Privacy policy|Modern Slavery Act Transparency Statement|Sitemap