Dynamic constrained optimization of chemical manufacturing
a technology of dynamic constrained optimization and chemical manufacturing, applied in the field of chemical production, can solve the problems of inability to achieve analytical solutions, the generality of the real world system precludes the possibility of achieving such solutions analytically, and the limitations of conventional computer fundamental models, so as to achieve the maximum feed to each section
- Summary
- Abstract
- Description
- Claims
- Application Information
AI Technical Summary
Benefits of technology
Problems solved by technology
Method used
Image
Examples
Embodiment Construction
Incorporation by Reference
[0081] U.S. application Ser. No. 09 / 827,838 titled “System and Method for Enterprise Modeling, Optimization and Control” and filed Apr. 5, 2001, whose inventors are Edward Stanley Plumer, Bijan Sayyar-Rodsari, Carl Anthony Schweiger, Ralph Bruce Ferguson II, William Douglas Johnson, and Celso Axelrud, is hereby incorporated by reference as though fully and completely set forth herein.
[0082] U.S. application Ser. No. 10 / 225,093 titled “System and Method for Real-Time Enterprise Optimization” and filed Aug. 21, 2002, whose inventors are Robert S. Golightly, John P. Havener, Ray D. Johnson, James D. Keeler and Ralph B. Ferguson, is hereby incorporated by reference as though fully and completely set forth herein.
Terms
[0083] Capacity—Capacity is the established maximum production rate of the process or unit under best operating conditions (no abnormal constraints). Capacity is a constant within the present capital investment. For new units it is the vendor...
PUM
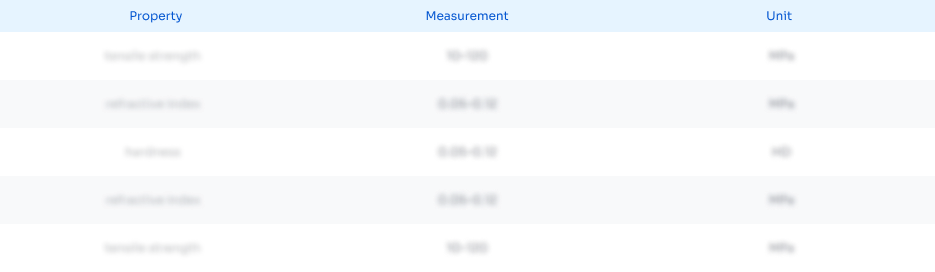
Abstract
Description
Claims
Application Information
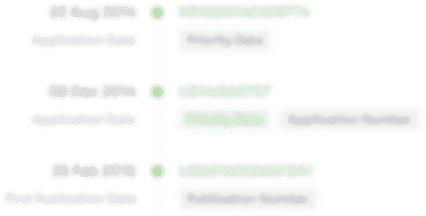
- R&D
- Intellectual Property
- Life Sciences
- Materials
- Tech Scout
- Unparalleled Data Quality
- Higher Quality Content
- 60% Fewer Hallucinations
Browse by: Latest US Patents, China's latest patents, Technical Efficacy Thesaurus, Application Domain, Technology Topic, Popular Technical Reports.
© 2025 PatSnap. All rights reserved.Legal|Privacy policy|Modern Slavery Act Transparency Statement|Sitemap|About US| Contact US: help@patsnap.com