Device and method for training a normalizing flow
a normalizing flow and flow training technology, applied in the field of normalizing flow training, can solve the problems of complicated finding such a model, inability to train a normalizing flow, etc., to achieve easy computable, influence the ability of predicting, and high performance
- Summary
- Abstract
- Description
- Claims
- Application Information
AI Technical Summary
Benefits of technology
Problems solved by technology
Method used
Image
Examples
Embodiment Construction
[0088]Shown in FIG. 1 is an example embodiment of a first method (1) for training a normalizing flow in the form of a flow chart. The normalizing flow is configured to accept images as inputs and comprises convolutional layers. Preferably, the normalizing flow consists only of convolutional layers as layers. Preferably, the normalizing flow does not comprise coupling or masked convolution layers as are used in, e.g., Real NVP.
[0089]In a first step (101) a training image (xi) is determined. The image may preferably be determined from a computer-implemented database comprising images that the normalizing flow shall be trained with, e.g., a training dataset of training images. Alternatively, the image may also be determined from a sensor during operation of the sensor. For example, the sensor may record an image and the image may then be used directly as training image (xi) for the normalizing flow. Preferably, the training image (xi) is in the form of a three-dimensional tensor of a p...
PUM
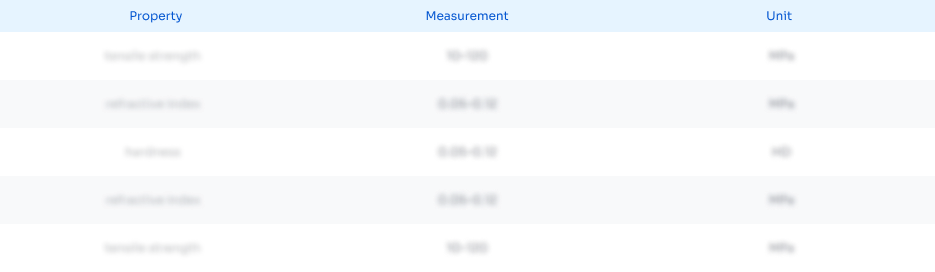
Abstract
Description
Claims
Application Information
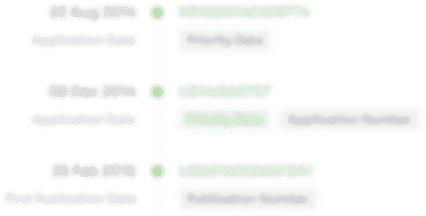
- R&D Engineer
- R&D Manager
- IP Professional
- Industry Leading Data Capabilities
- Powerful AI technology
- Patent DNA Extraction
Browse by: Latest US Patents, China's latest patents, Technical Efficacy Thesaurus, Application Domain, Technology Topic, Popular Technical Reports.
© 2024 PatSnap. All rights reserved.Legal|Privacy policy|Modern Slavery Act Transparency Statement|Sitemap|About US| Contact US: help@patsnap.com