Transportation data loss recovery method based on tensor reconstruction
A traffic data loss recovery technology, applied in the field of intelligent transportation
- Summary
- Abstract
- Description
- Claims
- Application Information
AI Technical Summary
Problems solved by technology
Method used
Image
Examples
Embodiment 1
[0061] This embodiment is aimed at the random loss of traffic data, according to figure 1 As shown, the recovery process is carried out in the following three steps:
[0062] 1. Organize the traffic data into a tensor form and mark the lost points
[0063] Since the traffic data in Figure 4(a) is 16-day traffic data, it can be formed as tensor traffic data by the following expression:
[0064] A∈R 16×12×24 (11)
[0065] Among them, A contains 16 days, 24 hours a day, 12 5 minutes an hour. According to the size of A is 16*12*24, mark the tensor The size is also 16*12*24, and the lost traffic data is determined by the following expression:
[0066] A'=Ω*A (12)
[0067] Among them, A represents the missing traffic data.
[0068] 2. Obtain the weight of each mode
[0069] For the specific flow chart of this process, please refer to figure 2 .includes the following steps:
[0070] First, expand the tensor traffic data to each mode. Because t...
Embodiment 2
[0082] For 10*12*24 traffic data, the tensor form is A∈R 10×12×24 , the corresponding tag tensor is The value of the tag tensor of the lost k days is determined according to the following expression:
[0083] in,
[0084] Among them, k represents the number of days lost, and then the lost traffic tensor data can be obtained.
[0085] The rest of the steps are the same as in Example 1. By calculating the weights of each model and bringing them into the objective function, and finally estimating the loss value, we get Figure 5 recovery effect. Figure 4 with Figure 5 The restoration effects in the method are all obtained under the Matlab environment. If the method of the present invention adopts C++ programming to realize, the running time will be greatly reduced, thereby realizing the automaticity and real-time performance of traffic data loss restoration.
PUM
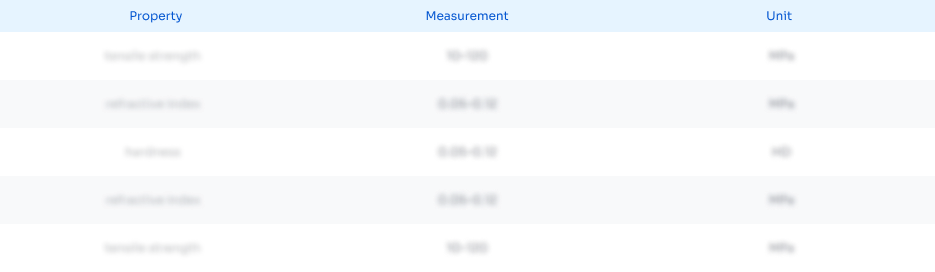
Abstract
Description
Claims
Application Information
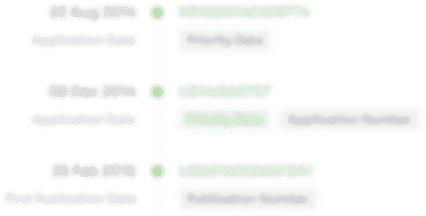
- R&D
- Intellectual Property
- Life Sciences
- Materials
- Tech Scout
- Unparalleled Data Quality
- Higher Quality Content
- 60% Fewer Hallucinations
Browse by: Latest US Patents, China's latest patents, Technical Efficacy Thesaurus, Application Domain, Technology Topic, Popular Technical Reports.
© 2025 PatSnap. All rights reserved.Legal|Privacy policy|Modern Slavery Act Transparency Statement|Sitemap|About US| Contact US: help@patsnap.com