Double-integration partial least square modeling method based on Monte Carlo and LASSO
A technique of least squares and modeling methods, applied in the field of analytical chemistry, can solve the problem of low accuracy of modeling and prediction, and achieve the effect of improving prediction ability and prediction accuracy
- Summary
- Abstract
- Description
- Claims
- Application Information
AI Technical Summary
Problems solved by technology
Method used
Image
Examples
Example Embodiment
[0040] Example 1:
[0041] This embodiment is applied to near-infrared spectroscopy data analysis to determine the oil content in corn samples. The specific steps are as follows:
[0042] 1) Collect 80 corn samples, use three different near-infrared spectrometers (M5, MP5, MP6) to measure the near-infrared spectroscopy data of corn, and use the oil content as the target value. The wavelength range of the near infrared spectrum is 2498~1100nm (4003~9091cm -1 ), the sampling interval is 2nm, a total of 700 wavelength data points. The data is downloaded from http: / / software .eigenvector.com / Data / Corn / index. html. Using the KS grouping method, 53 samples were used as the training set, and the remaining 27 samples were used as the prediction set. The near-infrared spectrum of the training set of this data is as figure 2 Shown.
[0043] 2) Determine the factor LV of the PLS model
[0044] Calculate the cross-validation root mean square error (RMSECV) under different factors, and th...
Example Embodiment
[0054] Example 2:
[0055] This embodiment is applied to the analysis of ultraviolet spectrum data to determine the content of monocyclic aromatic hydrocarbons in gasoline samples. The specific steps are as follows:
[0056] 1) Collect 115 light gasoline and diesel fuel samples, the ultraviolet spectrum wavelength range is 200-400nm, the sampling interval is about 0.35nm, a total of 572 wavelength data points. The content of monocyclic aromatic hydrocarbons was determined by HP model G1205A supercritical fluid chromatography (Hewlett-Packard, Palo Alto, Calif). The data is downloaded from http: / / myweb.dal.ca / pdwentze / downloads.html. The training set and prediction set are divided according to the instructions on the Internet. The first 70 samples are used as the training set, and the last 44 samples are used as the prediction set. The training set UV spectrum of this data is as Image 6 Shown.
[0057] 2) Determine the factor LV of the PLS model
[0058] Calculate the cross-valida...
Example Embodiment
[0068] Example 3:
[0069] This embodiment is applied to near-infrared spectroscopy data analysis to determine the content of sesame oil in a quaternary blend oil sample. The specific steps are as follows:
[0070] 1) Collect 51 quaternary blended oil samples containing sesame oil, corn oil, soybean oil and rice oil. Use Vertex70 multi-band infrared / near-infrared spectrometer (Bruker, Germany) for near-infrared spectroscopy data measurement, the wave number range is 4000~12000cm -1 , The sampling interval is 1.93cm -1 , A total of 4148 data points. Set the sesame oil content as the target value. Using the KS grouping method, 34 samples were used as the training set, and the remaining 17 samples were used as the prediction set. The near-infrared spectrum of the training set of this data is as Picture 10 Shown.
[0071] 2) Determine the factor LV of the PLS model
[0072] Calculate the cross-validation root mean square error (RMSECV) under different factors, and the factor correspo...
PUM
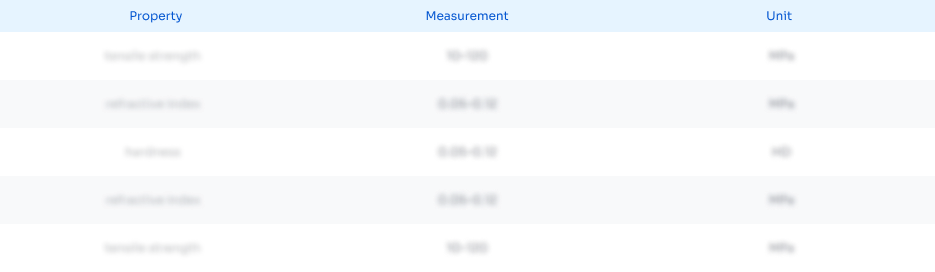
Abstract
Description
Claims
Application Information
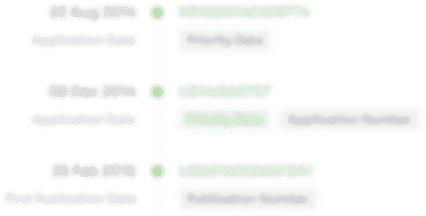
- R&D Engineer
- R&D Manager
- IP Professional
- Industry Leading Data Capabilities
- Powerful AI technology
- Patent DNA Extraction
Browse by: Latest US Patents, China's latest patents, Technical Efficacy Thesaurus, Application Domain, Technology Topic.
© 2024 PatSnap. All rights reserved.Legal|Privacy policy|Modern Slavery Act Transparency Statement|Sitemap