Distributed compression sensing-based hyperspectral image compression method
A hyperspectral image and compressed sensing technology, applied in the field of hyperspectral image compression, can solve the problems of insufficient redundancy removal, serious block effect of reconstructed images, high calculation amount and complexity, and achieve enhanced anti-error performance and improved performance. Accuracy, Effectiveness of Protecting Useful Information
- Summary
- Abstract
- Description
- Claims
- Application Information
AI Technical Summary
Problems solved by technology
Method used
Image
Examples
Embodiment 1
[0090] like figure 1 As shown, the present embodiment provides a hyperspectral image compression method based on distributed compressed sensing, the method comprising:
[0091] Step 01: For the hyperspectral image to be processed, based on the correlation of each band in the hyperspectral image and the information entropy value of each band, divide all the bands of the hyperspectral image into a low-correlation band group and multiple high-correlation bands Groups, each high-correlation band group includes: a reference band and multiple non-reference bands;
[0092] For example, this step 01 may include:
[0093] 011. Obtain information entropy values of all bands in the hyperspectral image; form bands corresponding to information entropy values greater than the first preset entropy value into a first set s 1 ;
[0094] 012. Obtain the correlation coefficient r between all adjacent bands in the hyperspectral image; determine two bands corresponding to each correlation c...
Embodiment 2
[0130] The traditional entropy method is used to select bands with rich information. Usually these bands are treated specially, for example, in prediction-based techniques like Differential Pulse Modulation (DPCM), the selected bands are usually used as reference bands to predict other non-reference bands. The prediction accuracy will be affected by the strength of correlation between the selected band and other bands in the group. If the band selected according to the entropy method has little correlation with the remaining bands in the group, the corresponding prediction error will be large. . From the analysis of the characteristics of hyperspectral images, the correlation and entropy curves between adjacent bands of hyperspectral images have similar trends, but the correlation curves and entropy curves do not completely coincide, and even appear opposite at the turning point of the curves. Trend, that is to say, when a band has a large entropy value, the correlation with ...
Embodiment 3
[0200] The superiority of this embodiment is described below in conjunction with experimental data and experimental results:
[0201] Two hyperspectral images of Terrain and Cuprite were selected as objects in the experiment, DWT was used as the sparse basis, the random observation matrix was a partial Hadamard matrix, and the CS reconstruction method was the Basis Pursuit (BP) method.
[0202]The band selection results remain unchanged, and the grouping conditions are shown in Table 1 and Table 2. Sampling rate SR=M / N×100%, M is the number of rows of the observation matrix, and N is the length of the original signal after sparse. Under the same conditions (Intel single-core 2.66GHz / 32-bit operating system memory 2GB), in this embodiment, the 3D-SPIHT algorithm, the IOI-DCS algorithm (the algorithm using fixed band grouping) and the CE-DCS algorithm are compared.
[0203] Table 3 and Table 4 respectively give the average peak signal-to-noise ratio (Average PSNR, APSNR) of the...
PUM
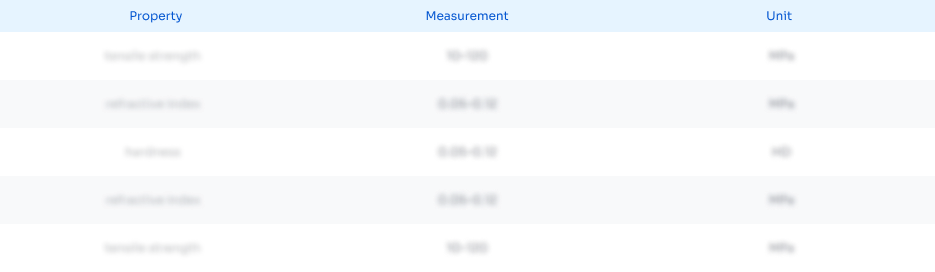
Abstract
Description
Claims
Application Information
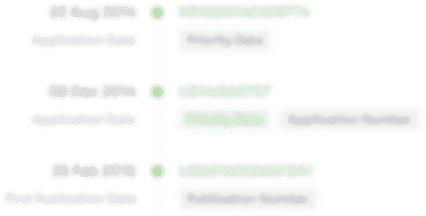
- R&D Engineer
- R&D Manager
- IP Professional
- Industry Leading Data Capabilities
- Powerful AI technology
- Patent DNA Extraction
Browse by: Latest US Patents, China's latest patents, Technical Efficacy Thesaurus, Application Domain, Technology Topic.
© 2024 PatSnap. All rights reserved.Legal|Privacy policy|Modern Slavery Act Transparency Statement|Sitemap