Deep learning algorithm-based quantitative modeling method of near-infrared spectroscopy of tobacco and model application
A technology of near-infrared spectroscopy and deep learning, which is applied in the field of quantitative modeling of near-infrared spectroscopy for the analysis and prediction of tobacco leaf chemical components, can solve the problems of inability to extract useful information, low precision, overlapping spectral peaks, etc., and achieve improved quantitative construction. Die Efficiency Effect
- Summary
- Abstract
- Description
- Claims
- Application Information
AI Technical Summary
Problems solved by technology
Method used
Image
Examples
Embodiment Construction
[0018] The present invention will be described in further detail below in conjunction with the accompanying drawings and specific embodiments, but the protection scope of the present invention is not limited to the content described.
[0019] Quantitative modeling method of near-infrared spectrum of tobacco leaves based on deep learning, such as figure 1 shown, including the following steps:
[0020] ① Obtain the near-infrared spectral information of tobacco leaves, and perform preprocessing operations on the spectral data. In this embodiment, the existing near-infrared spectrometer is used to collect spectral information, and the collection range is between 1000 nm and 2500 nm wavelength or any part thereof. The preprocessing of spectral information includes eliminating baseline drift and removing spectral noise; spectral information preprocessing methods include wavelet transform algorithm, SG convolution smoothing method, multivariate scattering correction method, first-or...
PUM
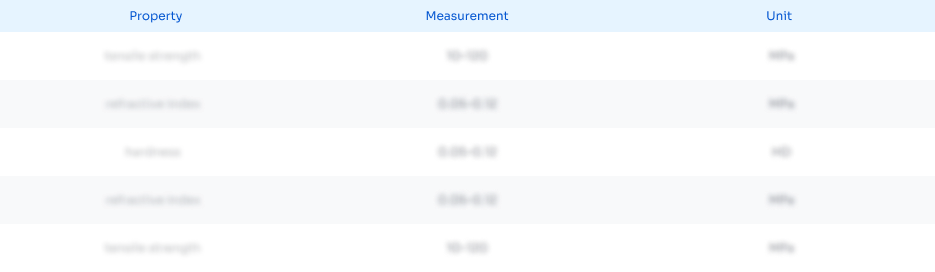
Abstract
Description
Claims
Application Information
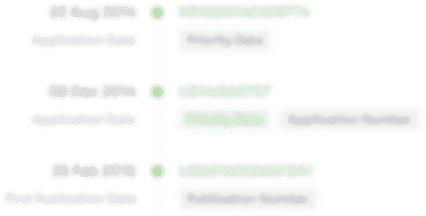
- R&D Engineer
- R&D Manager
- IP Professional
- Industry Leading Data Capabilities
- Powerful AI technology
- Patent DNA Extraction
Browse by: Latest US Patents, China's latest patents, Technical Efficacy Thesaurus, Application Domain, Technology Topic, Popular Technical Reports.
© 2024 PatSnap. All rights reserved.Legal|Privacy policy|Modern Slavery Act Transparency Statement|Sitemap|About US| Contact US: help@patsnap.com