Personalized real-time recommendation method for online learning resources
A learning resource and real-time recommendation technology, applied in the field of e-learning, can solve the problems of high algorithm complexity, inappropriate online recommendation, and low recommendation quality.
- Summary
- Abstract
- Description
- Claims
- Application Information
AI Technical Summary
Problems solved by technology
Method used
Image
Examples
Embodiment Construction
[0079] In order to make the technical means and effects realized by the present invention easy to understand, the present invention will be described in detail below in conjunction with the embodiments and accompanying drawings.
[0080]
[0081] figure 1 It is a flowchart of a method for personalized real-time recommendation of network online learning resources in an embodiment of the present invention.
[0082] Such as figure 1 As shown, the personalized real-time recommendation method of online learning resources can personalize learning resources to learners according to the knowledge points selected by the learners in the online learning system through the input display part of the user terminal and the self-cognition ability input. Real-time recommendation, including the following steps:
[0083] Step 1. Obtain the learner's learning feature parameters and the course feature parameters of the recommended course recommended to the learner from the online learning syst...
PUM
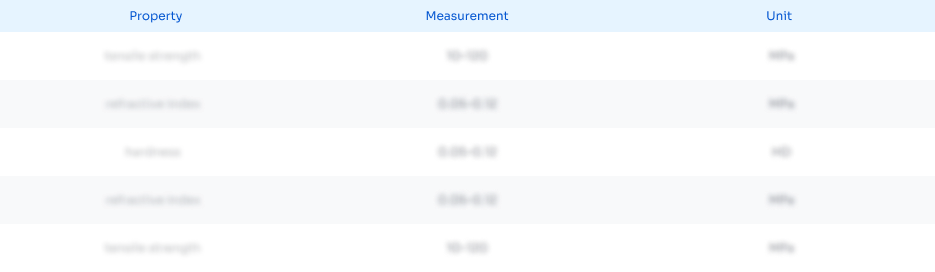
Abstract
Description
Claims
Application Information
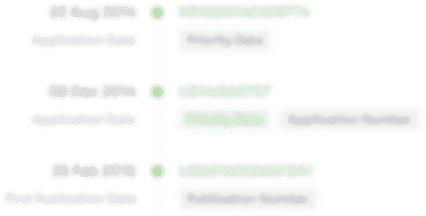
- R&D Engineer
- R&D Manager
- IP Professional
- Industry Leading Data Capabilities
- Powerful AI technology
- Patent DNA Extraction
Browse by: Latest US Patents, China's latest patents, Technical Efficacy Thesaurus, Application Domain, Technology Topic.
© 2024 PatSnap. All rights reserved.Legal|Privacy policy|Modern Slavery Act Transparency Statement|Sitemap