Self-adapting method of DNN acoustic model based on personal identity characteristics
An acoustic model and identity feature technology, applied in the field of communication, can solve the problems of mismatch between the speaker's voice of the training data and the target speaker's voice, reducing the accuracy of DNN frame classification, and unable to make full use of speaker information, etc., to improve the system. Recognition performance, overcoming the drop in accuracy, and good adaptive performance
- Summary
- Abstract
- Description
- Claims
- Application Information
AI Technical Summary
Problems solved by technology
Method used
Image
Examples
Embodiment 1
[0034]In recent years, more and more attention has been paid to speaker adaptive technology. Adaptive methods are divided into model domain adaptive and feature domain adaptive. The application of adaptive technology in hidden Markov-Gaussian mixture HMM-GMM systems has been widely used. It is mature, but it is difficult to directly apply it to the hidden Markov-deep neural network HMM-DNN system. Many research institutions have done a lot of research on the adaptation of deep neural networks. Among these methods, speaker adaptation based on i-vector is very popular. However, the current adaptive research methods have not made full use of a small amount of adaptive data, and the network structure is complex, the calculation complexity is high, and the stability is not good enough. The present invention just researches and discusses the i-vector adaptive method based on the deep neural network DNN, and proposes an adaptive method based on the DNN acoustic model of the personal...
Embodiment 2
[0048] The adaptive method of the DNN acoustic model based on the personal identity (i-vector) feature is the same as embodiment 1, and the extraction of the personal identity i-vector feature described in step 1 of the present invention includes the following steps
[0049] 1a) Utilize the 39-dimensional low-dimensional feature MFCC extracted from the speech data of the test set in the open source corpus, including its first-order and second-order features, and train a DNN model for non-specific speaker feature extraction;
[0050] 1b) Apply the singular value matrix decomposition technique SVD to decompose the last hidden layer weight matrix of the DNN model trained in step 1a) for non-specific speaker feature extraction, and use it to replace the original weight matrix.
[0051] 1c) Apply the backpropagation algorithm (BP) and the gradient descent method to train the DNN model, and then use the trained DNN model to extract low-dimensional features of non-specific speakers. ...
Embodiment 3
[0055] The adaptive method of the DNN acoustic model based on personal identity (i-vector) feature is the same as embodiment 1-2, the extraction of the characteristic speaker identity vector i-vector described in (1c) and (1d) in the step, its expression for:
[0056] M=m+Tx+e
[0057] where M denotes the speaker-specific GMM mean supervector, m denotes the UBM mean supervector, T denotes a total feature space, x denotes the extracted i-vector features representing personal identity, and e denotes the residual noise term.
[0058] In this example, based on the training data in the corpus, it is easy to obtain the general background model UBM, and the total change matrix T is obtained through the expectation maximization (EM) algorithm. The personal identity i-vcetor feature extracted by this method has a good speaker distinction , which represents the difference information between speakers, and has the advantages of low dimensionality, few parameters during adaptive training...
PUM
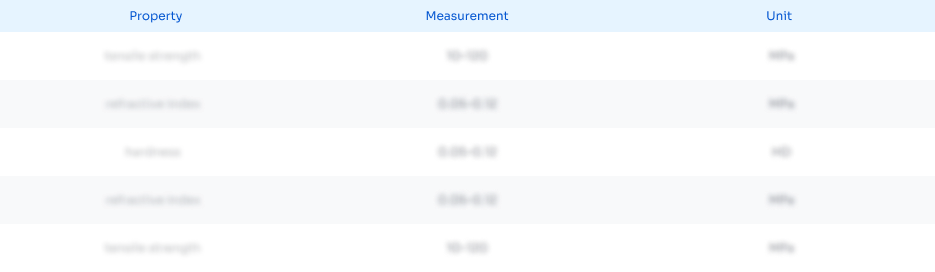
Abstract
Description
Claims
Application Information
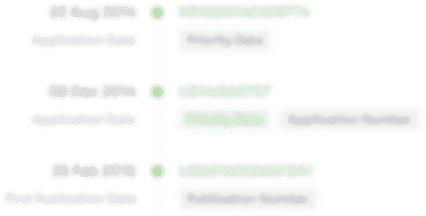
- Generate Ideas
- Intellectual Property
- Life Sciences
- Materials
- Tech Scout
- Unparalleled Data Quality
- Higher Quality Content
- 60% Fewer Hallucinations
Browse by: Latest US Patents, China's latest patents, Technical Efficacy Thesaurus, Application Domain, Technology Topic, Popular Technical Reports.
© 2025 PatSnap. All rights reserved.Legal|Privacy policy|Modern Slavery Act Transparency Statement|Sitemap|About US| Contact US: help@patsnap.com