An Abnormal Traffic Detection Method Based on Autoencoder Network
An autoencoder and abnormal traffic technology, applied in the direction of neural learning methods, biological neural network models, electrical components, etc., can solve the problem of unbalanced distribution of normal traffic data and intrusion data, the inability to predict different attack types of intrusion traffic, and no Taking into account the unbalanced data distribution and other issues, it achieves the effect of excellent expressive ability, small footprint, and self-learning expressive ability
- Summary
- Abstract
- Description
- Claims
- Application Information
AI Technical Summary
Problems solved by technology
Method used
Image
Examples
Embodiment Construction
[0022] Embodiments of the present invention will be described in detail below.
[0023] An abnormal traffic detection method based on autoencoder network, refer to figure 2 , the method is implemented based on four modules: traffic data acquisition module, data preprocessing module, hash coding module based on autoencoder network, and anomaly detection module, specifically:
[0024] (1) Traffic data acquisition module
[0025] Finding out valuable available data information is the first step to realize intrusion detection, and it is also the key of the whole intrusion detection. The data information collected by the intrusion detection system mainly comes from different network segments or hosts. Among them, the user activity status and network system are the main data sources. In order to maximize the effectiveness of the detection system and make fast and accurate attacks Response, which requires data information to have a certain degree of reliability. In this module, s...
PUM
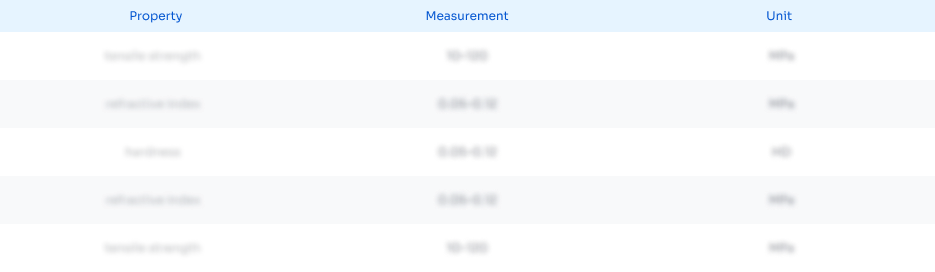
Abstract
Description
Claims
Application Information
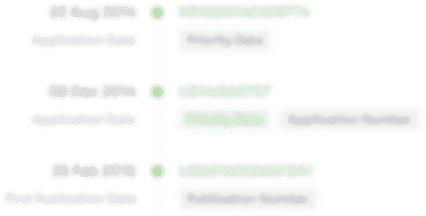
- R&D
- Intellectual Property
- Life Sciences
- Materials
- Tech Scout
- Unparalleled Data Quality
- Higher Quality Content
- 60% Fewer Hallucinations
Browse by: Latest US Patents, China's latest patents, Technical Efficacy Thesaurus, Application Domain, Technology Topic, Popular Technical Reports.
© 2025 PatSnap. All rights reserved.Legal|Privacy policy|Modern Slavery Act Transparency Statement|Sitemap|About US| Contact US: help@patsnap.com