Unsupervised monocular view depth estimation method based on multi-scale unification
A depth estimation, multi-scale technology, applied in the field of image processing, can solve the problems of lack of depth map texture, affecting depth estimation accuracy, holes, etc., to improve the depth map holes, solve the depth map holes, and reduce the difficulty of acquisition.
- Summary
- Abstract
- Description
- Claims
- Application Information
AI Technical Summary
Problems solved by technology
Method used
Image
Examples
Embodiment Construction
[0043] The following will clearly and completely describe the technical solutions in the embodiments of the present invention with reference to the accompanying drawings in the embodiments of the present invention. Obviously, the described embodiments are only some, not all, embodiments of the present invention.
[0044] refer to Figure 1-6 , an unsupervised monocular depth estimation method based on multi-scale unification, in which the unsupervised depth monocular depth estimation network model is carried out on the desktop workstation of this laboratory, the graphics card uses NVIDIA GeForceGTX 1080Ti, and the training system is Ubuntu14.04. TensorFlow 1.4.0 is used as the framework to build the platform; training is carried out on the classic driving data set KITTI 2015 stereo data set.
[0045] Such as figure 1 As shown, an unsupervised monocular view depth estimation method based on multi-scale unity of the present invention specifically includes the following steps: ...
PUM
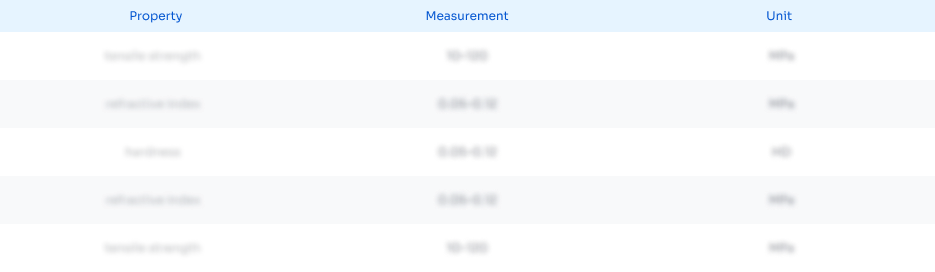
Abstract
Description
Claims
Application Information
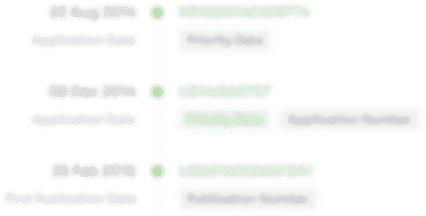
- R&D Engineer
- R&D Manager
- IP Professional
- Industry Leading Data Capabilities
- Powerful AI technology
- Patent DNA Extraction
Browse by: Latest US Patents, China's latest patents, Technical Efficacy Thesaurus, Application Domain, Technology Topic, Popular Technical Reports.
© 2024 PatSnap. All rights reserved.Legal|Privacy policy|Modern Slavery Act Transparency Statement|Sitemap|About US| Contact US: help@patsnap.com