Pneumonia picture calibrating device and method capable of self-adaptively adjusting size of receptive field
A technology of self-adaptive adjustment and receptive field, applied in the field of medical equipment and deep learning convolutional neural network, can solve the problems of high model complexity, slow detection speed, low result accuracy, etc., to improve the detection speed, reduce the burden, improve the The effect of efficiency
- Summary
- Abstract
- Description
- Claims
- Application Information
AI Technical Summary
Problems solved by technology
Method used
Image
Examples
Embodiment Construction
[0038] With the increasing number of patients with pneumonia, the problem of insufficient time and energy for radiologists to diagnose X-rays has gradually become prominent. The use of neural networks can save manpower and quickly detect the location of pneumonia lesions. However, since pneumonia shows increased opacity in X-ray films, it cannot be clearly judged from the appearance, so the discrimination of neural networks is very limited when extracting features, resulting in insufficient accuracy of the results. high. Increasing the number of network layers will increase time complexity and reduce efficiency. Therefore, how to use the neural network to improve the detection accuracy without excessively increasing the network complexity has become an urgent problem to be solved.
[0039]The overall technical solution of the present invention is: the whole is roughly divided into three parts: feature extraction network, feature pyramid, classification sub-branch and regressi...
PUM
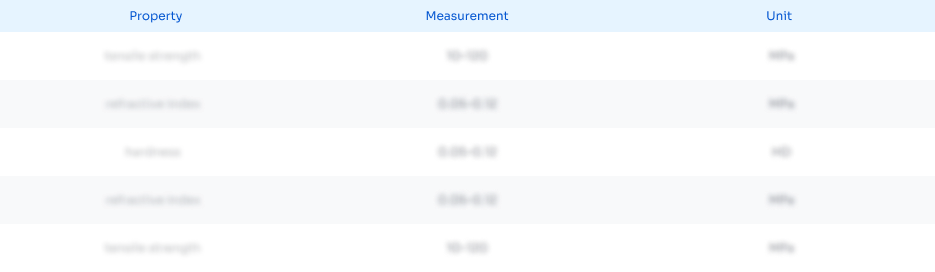
Abstract
Description
Claims
Application Information
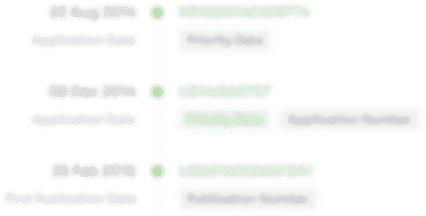
- R&D Engineer
- R&D Manager
- IP Professional
- Industry Leading Data Capabilities
- Powerful AI technology
- Patent DNA Extraction
Browse by: Latest US Patents, China's latest patents, Technical Efficacy Thesaurus, Application Domain, Technology Topic, Popular Technical Reports.
© 2024 PatSnap. All rights reserved.Legal|Privacy policy|Modern Slavery Act Transparency Statement|Sitemap|About US| Contact US: help@patsnap.com