Adiabatic acceleration calorimetric method based on machine learning
A technology of accelerated calorimetry and machine learning, applied in the direction of thermal development of materials, etc., can solve the problems of temperature tracking process hysteresis and other problems
- Summary
- Abstract
- Description
- Claims
- Application Information
AI Technical Summary
Problems solved by technology
Method used
Image
Examples
Embodiment 1
[0040] A machine learning-based adiabatic accelerated calorimetry method, which is based on a mathematical nonlinear exponential growth fitting formula without any physical and chemical parameters, combined with the initially known data of the exothermic stage of the adiabatic process of the measured sample, the fitting is obtained to describe the adiabatic The fitting curve and fitting formula of exothermic reaction; and then carry out repeated experiments, so that the temperature control system of the instrument can be programmed to increase the temperature according to the fitting formula to obtain an adiabatic reaction curve that is more in line with the actual situation. The temperature difference between the experimental curves obtained by carrying out repeated experiments according to the formula, when the difference is small enough (ΔT≤1°C), it is judged that the obtained experimental curve is the closest to the actual adiabatic heat release process of the sample, which ...
Embodiment 2
[0054] This embodiment provides a method of adiabatic acceleration calorimetry based on machine learning, which is mainly applied to the calorimetric test of the adiabatic heat release stage of the sample in the heating-waiting-search mode. The heating-waiting-searching mode is the main function of the adiabatic acceleration calorimeter. The operation mode is the most widely used, so this embodiment can meet the requirements of different security detection and risk assessment.
[0055] A method of adiabatic accelerated calorimetry based on machine learning. The method takes the selected mathematical function model as the core. Since the chemical reaction system is exothermic during the thermal runaway process and the exothermic rate gradually accelerates with the increase of the reaction temperature, it shows Exponential growth trend, and the chemical reaction system has a certain complexity, so this embodiment chooses a second-order exponential growth fitting function to descr...
Embodiment 3
[0074] The decomposition process of organic peroxides is a typical exothermic reaction, and the decomposition rate is from slow to fast. When the temperature rises to a certain level, autothermal decomposition will occur, which may eventually lead to thermal runaway or more serious hazards; this example uses Taking tert-butyl hydroperoxide (TBHP) standard solution as an example, the adiabatic exothermic process of TBHP was explored according to the adiabatic acceleration calorimeter experiment, so as to achieve better thermal and kinetic analysis and evaluation.
[0075] The specific analysis process is as follows: the adiabatic acceleration calorimeter is selected as the heating-waiting-search (H-W-S) mode, the temperature in the start-up interval is set to 70°C, the constant temperature time in the start-up interval is 40min, the step heating rate is 10°C / min, and the step length of the step temperature rise is 5°C, the temperature detection threshold is 0.02°C / min, and the s...
PUM
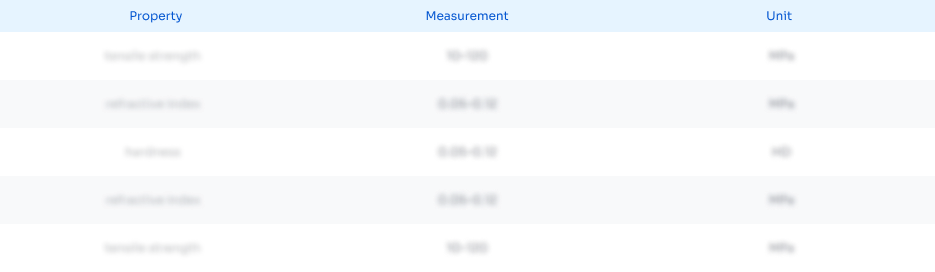
Abstract
Description
Claims
Application Information
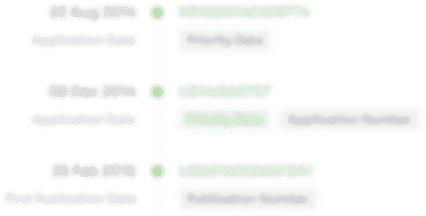
- R&D Engineer
- R&D Manager
- IP Professional
- Industry Leading Data Capabilities
- Powerful AI technology
- Patent DNA Extraction
Browse by: Latest US Patents, China's latest patents, Technical Efficacy Thesaurus, Application Domain, Technology Topic, Popular Technical Reports.
© 2024 PatSnap. All rights reserved.Legal|Privacy policy|Modern Slavery Act Transparency Statement|Sitemap|About US| Contact US: help@patsnap.com