Complex radar radiation source identification method based on one-dimensional self-stepping convolutional neural network
A technology of convolutional neural network and recognition method, which is applied in radar radiation source recognition, complex radar radiation source recognition field based on one-dimensional self-paced convolutional neural network, can solve the problem of suppressing radar radiation source classification and recognition accuracy, low recognition rate, It takes a lot of time and other problems to achieve the effect of superior real-time performance, simple network structure and high recognition accuracy
- Summary
- Abstract
- Description
- Claims
- Application Information
AI Technical Summary
Problems solved by technology
Method used
Image
Examples
Embodiment 1
[0021]In today's electromagnetic environment, the radar system is constantly being updated, and the electronic environment is becoming more dense and complex. Effectively identifying radar radiation source signals with high accuracy is a difficult problem in today's electronic warfare, and it is also an important topic. It can not only improve the passive radar system, but also has great research value for the performance improvement of the active radar system. Many experts use two-dimensional convolutional neural networks to identify radar emitter signals. However, the structure of the traditional two-dimensional convolutional neural network is relatively complicated, and the dimensional transformation of the collected one-dimensional radar source signal is required. At the same time, the traditional two-dimensional convolutional neural network has poor recognition effect on radar radiation sources and low recognition accuracy. In view of the above problems, the present invention p...
Embodiment 2
[0033]The complex radar emitter identification method based on the one-dimensional self-stepping convolutional neural network is the same as the seven different modulation types of radar signals described in Example 1, step 1), and the corresponding parameters are set as follows:
[0034]The sampling frequency of these seven different modulation types of radar signals is set to 2GHz, and the number of sampling points is set to 1024;
[0035]The carrier frequency range of conventional pulse signals is 200-220MHz.
[0036]The carrier frequency range of the chirp signal is 200-220MHz, and the bandwidth range is 50-60MHz.
[0037]The nonlinear frequency modulation signal adopts cosine modulation, the carrier frequency range is 200-220MHz, and the modulation signal range is 10-12MHz;
[0038]The carrier frequency range of the two-phase encoded signal is 200-220MHz, the encoding method adopts 13-bit Barker code, the pulse width is 0.5us, and the symbol width is 0.038us.
[0039]The carrier frequency rang...
Embodiment 3
[0044]The complex radar emitter identification method based on the one-dimensional self-stepping convolutional neural network is the same as the construction of the one-dimensional self-stepping convolutional neural network described in Example 1-2, step 3), seefigure 2 , The specific network structure includes the following:
[0045]The first layer is the input layer, the number of nodes is 1024;
[0046]The second layer is a one-dimensional convolution layer with 32 convolution kernels and a convolution kernel size of 33;
[0047]The third layer is a pooling layer with a pooling window of 2, a step size of 2, and a sampling maximum pooling method;
[0048]The fourth layer is the batch normalization layer;
[0049]The fifth layer is a one-dimensional convolution layer with 32 convolution kernels and a convolution kernel size of 33;
[0050]The sixth layer is a pooling layer with a pooling window of 2, a step size of 2, and a maximum sampling pooling method;
[0051]The seventh layer is the batch normal...
PUM
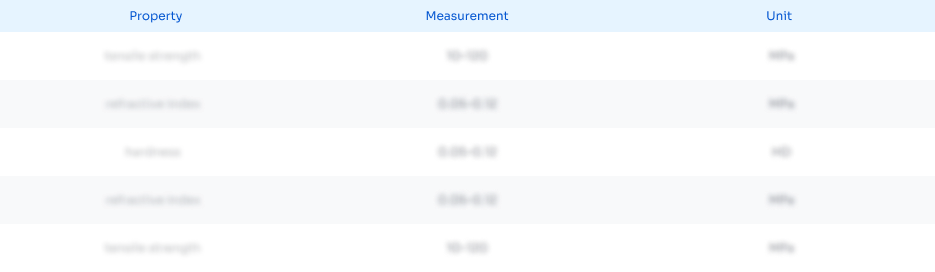
Abstract
Description
Claims
Application Information
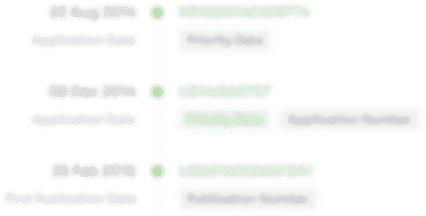
- R&D Engineer
- R&D Manager
- IP Professional
- Industry Leading Data Capabilities
- Powerful AI technology
- Patent DNA Extraction
Browse by: Latest US Patents, China's latest patents, Technical Efficacy Thesaurus, Application Domain, Technology Topic, Popular Technical Reports.
© 2024 PatSnap. All rights reserved.Legal|Privacy policy|Modern Slavery Act Transparency Statement|Sitemap|About US| Contact US: help@patsnap.com