Product quality control method based on process network model and machine learning algorithm
A machine learning and network model technology, applied in machine learning, computing models, instruments, etc., can solve problems such as comprehensive analysis from a global perspective, high-dimensional industrial big data, uneven distribution of strongly nonlinear samples, and small data processing volume. To achieve the effect of improving the product qualification rate
- Summary
- Abstract
- Description
- Claims
- Application Information
AI Technical Summary
Problems solved by technology
Method used
Image
Examples
Embodiment
[0085] In this embodiment, the key process parameters that affect the process quality of aviation turbine blade precision casting process are analyzed as an example, and the steps of the analysis are as follows by adopting the product quality control method based on the process network model and machine learning algorithm of the present invention:
[0086] Step 1. Sort out and confirm the process flow and quality parameters of precision casting turbine blades.
[0087] The precision casting turbine blade casting process includes 5 sections of core manufacturing, wax film manufacturing, coating shell, melting casting, and post-processing. Consider the influence of materials and processes in each section on the processing quality, sort out the procedures of the 5 sections and the key process parameters in each procedure, and confirm the intermediate inspection results of each procedure, such as Figure 6-10 . Integrating the entire precision casting blade processing process, th...
PUM
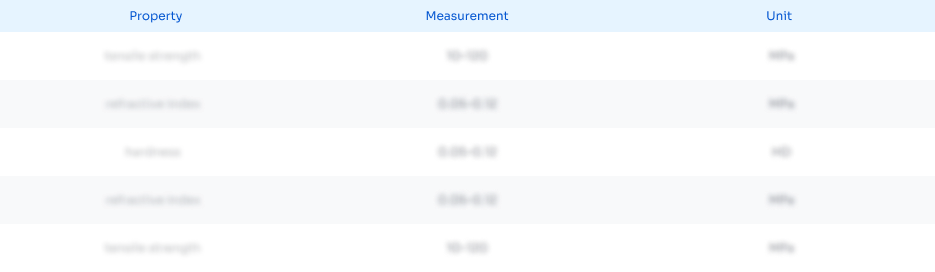
Abstract
Description
Claims
Application Information
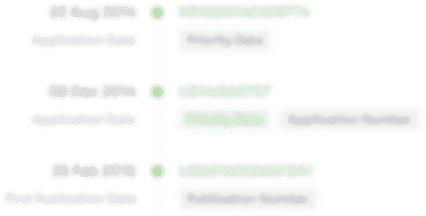
- R&D Engineer
- R&D Manager
- IP Professional
- Industry Leading Data Capabilities
- Powerful AI technology
- Patent DNA Extraction
Browse by: Latest US Patents, China's latest patents, Technical Efficacy Thesaurus, Application Domain, Technology Topic, Popular Technical Reports.
© 2024 PatSnap. All rights reserved.Legal|Privacy policy|Modern Slavery Act Transparency Statement|Sitemap|About US| Contact US: help@patsnap.com