Large-watershed runoff simulation method based on computer vision and LSTM neural network
A computer vision and neural network technology, applied in the field of large watershed runoff simulation, can solve the problems of difficult to effectively screen and make full use of data space information, unable to make full use of input data space characteristics, low model operation efficiency, etc. The effect of shortening operation time and improving operation efficiency
- Summary
- Abstract
- Description
- Claims
- Application Information
AI Technical Summary
Problems solved by technology
Method used
Image
Examples
Embodiment 1
[0072] Embodiment 1: a kind of large watershed runoff simulation method based on computer vision and LSTM neural network, concrete steps are as follows:
[0073] (1) Collect the meteorological and hydrological data sets of the watershed, in which the meteorological and hydrological data sets include the flow time series of the target section and the precipitation data of the whole watershed;
[0074] Meteorological and hydrological data sets can also include temperature, wind speed, humidity, solar radiation, snow cover, snow water equivalent, leaf area index (LAI), normalized difference vegetation index (NDVI), soil moisture, water storage (gravity One or more of satellite data derivation);
[0075] (2) standardize and normalize the meteorological and hydrological data set in step (1) to 0-1 to obtain the model input data set;
[0076] The data is standardized to a unified data resolution, and the normalization process is to normalize the original meteorological and hydrolog...
Embodiment 2
[0130] Embodiment 2: In this embodiment, runoff simulation is performed in the Ayeyarwady River Basin;
[0131] Overview of the Ayeyarwady River Basin: The Ayeyarwady River flows through China, India, and Myanmar. The basin covers about 60% of Myanmar's territory, and more than 90% of Myanmar's population lives there. It is known as the mother river of Myanmar. The Ayeyarwady River originates in Zayu County, Tibet, China. Its main tributaries include Daying River, Ruili River, Qindun River, Mu River, Yao River and Meng River. 430,000km 2 ;
[0132] A large watershed runoff simulation method based on computer vision and LSTM neural network, the specific steps are as follows:
[0133] (1) Collect the meteorological and hydrological data sets of the basin. According to the data availability of the Ayeyarwady River basin, collect the daily flow time series of the PYAY station of the control section of the basin from 1996 to 2010, and the model input data set of the whole basin f...
PUM
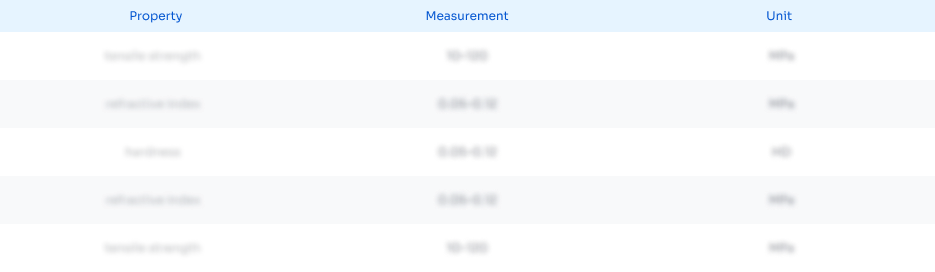
Abstract
Description
Claims
Application Information
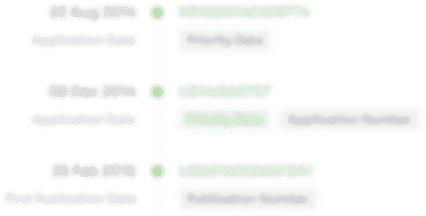
- R&D Engineer
- R&D Manager
- IP Professional
- Industry Leading Data Capabilities
- Powerful AI technology
- Patent DNA Extraction
Browse by: Latest US Patents, China's latest patents, Technical Efficacy Thesaurus, Application Domain, Technology Topic, Popular Technical Reports.
© 2024 PatSnap. All rights reserved.Legal|Privacy policy|Modern Slavery Act Transparency Statement|Sitemap|About US| Contact US: help@patsnap.com