Low-dose CT (Computed Tomography) image denoising method and system based on gradient guidance and self-similarity correction
A self-similarity, CT image technology, applied in the field of image processing, can solve the problems of distortion, denoising image texture details, smooth structure, large computing resource consumption, etc., achieve simple and accurate calculation, alleviate model parameter redundancy, and improve utilization. rate effect
- Summary
- Abstract
- Description
- Claims
- Application Information
AI Technical Summary
Problems solved by technology
Method used
Image
Examples
Embodiment 1
[0046] Such as figure 1 As shown, the present embodiment provides a low-dose CT image denoising method guided by gradient and self-similarity correction, comprising the following steps:
[0047] S1. Obtain the noisy gradient image of the first image according to the gradient extraction operator, which is denoted as the first noisy gradient image; extract the shallow features of the first image and the first noisy gradient image, respectively denoted as the shallow layer of the first image features and first gradient shallow features;
[0048] S2, performing m times of gradient guidance and self-similarity correction;
[0049] Among them, the steps of each gradient guidance and self-similar correction are as follows:
[0050] Input the first image feature and the first gradient feature; if the gradient guidance and self-similar correction are performed for the first time, the first image feature is the first image shallow feature; the first gradient feature is the first gradi...
Embodiment 2
[0074] This embodiment describes in detail the gradient-guided and self-similarity-corrected low-dose CT image denoising method described in Embodiment 1 based on the application field of low-dose CT images. The network structure diagram of the denoising method is as follows figure 2 As shown, the size of the convolution kernel except the last layer is 3×3, the size of the convolution kernel of the last layer is 1×1, the encoding module is composed of a large-step convolution layer, and the decoding module is a deconvolution layer. The flowchart of the denoising method is shown in image 3 As shown, the specific steps are as follows:
[0075] Specifically, in step S1, the first image is a low-dose CT image; the low-dose CT image is expressed as Where H is the image height and W is the image width. build as Figure 4 The gradient extraction operator shown is used to obtain low-dose CT images according to the gradient extraction operator The noisy gradient image of is de...
Embodiment 3
[0110] Such as Figure 8 As shown, this embodiment provides a low-dose CT image denoising system guided by gradient and self-similarity correction, including a first image shallow feature extraction module, a plurality of first components, a feature reconstruction module, a gradient extraction module, The first gradient shallow feature extraction module, multiple second components and gradient feature reconstruction module; the first image is respectively input to the gradient extraction module and the first image shallow feature extraction module; the gradient extraction module is extracted through the first gradient shallow feature extraction module, a plurality of second components are connected to the gradient feature reconstruction module; the second component includes the first gradient encoding module and the first gradient decoding module connected in sequence; the first image shallow feature extraction module is passed through a plurality of first A component is conne...
PUM
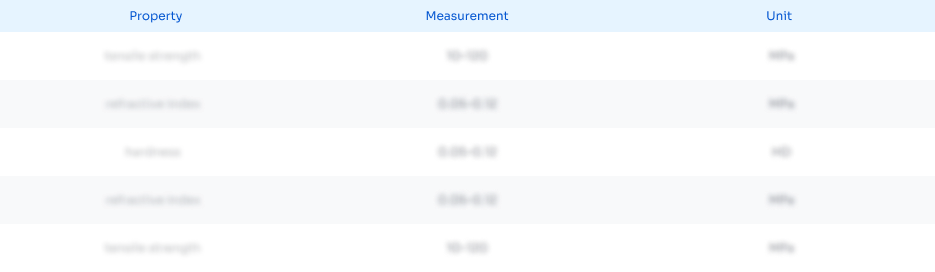
Abstract
Description
Claims
Application Information
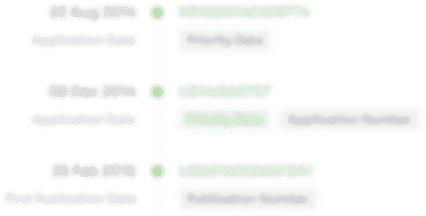
- R&D Engineer
- R&D Manager
- IP Professional
- Industry Leading Data Capabilities
- Powerful AI technology
- Patent DNA Extraction
Browse by: Latest US Patents, China's latest patents, Technical Efficacy Thesaurus, Application Domain, Technology Topic, Popular Technical Reports.
© 2024 PatSnap. All rights reserved.Legal|Privacy policy|Modern Slavery Act Transparency Statement|Sitemap|About US| Contact US: help@patsnap.com