Tailing activation process system based on drying, grinding and selecting integrated intelligent production equipment
An intelligent production and tailings technology, applied in cement production, grain processing, etc., can solve problems such as activity index fluctuations, process cannot be monitored in real time, and the cost of grinding powder is increased, so as to achieve the effect of high quality and efficient production
- Summary
- Abstract
- Description
- Claims
- Application Information
AI Technical Summary
Problems solved by technology
Method used
Image
Examples
Embodiment 1
[0064] figure 1 A layout mode of the tailings activation process system based on the integrated intelligent production equipment of drying, grinding and separating is given, including an intelligent "drying, grinding and separating" integrated machine A, and the intelligent system connected by setting a closed channel D of a conveyor belt. Chemical activation machine B, and intelligent production execution system C for comprehensive utilization of tailings.
[0065] Among them, the structure of the intelligent "drying, grinding and selecting" all-in-one machine A is as follows: figure 2 As shown, it includes: an outer frame 1, a mill composed of a vibration-assisted multi-layer grinding mechanism and a suspended mill box, a multi-point air intake drying device 5, and a circulating powder selection system, wherein the suspended mill The box is divided into an upper box body 2 and a lower box body 3. The lower box body 3 is flexibly connected with the outer frame 1 by an elast...
Embodiment 2
[0077] Such as Figure 5 As shown, the ELM algorithm for real-time monitoring of equipment failures specifically includes:
[0078] S1 collects the abnormal data of intelligent "drying, grinding and selecting" all-in-one machine and intelligent activation machine, establishes the mapping relationship between faults and abnormal data characteristic parameters after analysis, and forms a fault database;
[0079] S2 conducts off-line big data analysis and feature extraction on abnormal data; finally, calculates through the ELM extreme learning algorithm, and compares the calculation results with the fault database to determine the type of equipment fault.
[0080] Among them, S1 specifically includes:
[0081] The second module described in S1-1 is respectively used for the first current sensor, the first voltage sensor, the first displacement sensor, the high-temperature airflow temperature sensor, the first tension sensor, the first vibration frequency sensor, the infrared sen...
Embodiment 3
[0091] The LSTM algorithm monitors the energy consumption of the system in real time, including:
[0092] The second module of P1 collects the data of the first current sensor, the first voltage sensor, the second current sensor, and the second voltage sensor in real time, and obtains multiple energy consumption time series data C during the same time period in consecutive days i (t), i∈[1,90], the number of samples is 90, and it is divided into training set and verification set, and the ratio of the two is 4:1;
[0093] P2 as Figure 7 As shown, build the LSTM model and choose the initial time t 0 and the target time t g Energy consumption time series data for periods between The initial energy consumption C i (t 0 ), energy consumption C at each time node i (t j ) Energy consumption C at the target moment of the sequence i (t g ) into the LSTM model to get the predicted energy consumption at each time node Figure 7 Contains the cell state C that is transmitte...
PUM
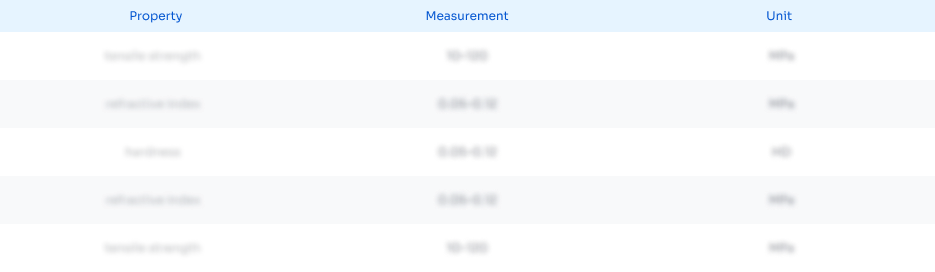
Abstract
Description
Claims
Application Information
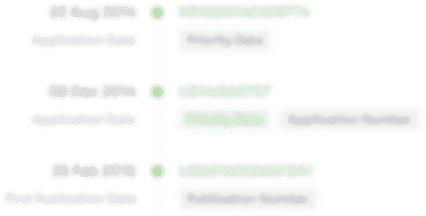
- R&D Engineer
- R&D Manager
- IP Professional
- Industry Leading Data Capabilities
- Powerful AI technology
- Patent DNA Extraction
Browse by: Latest US Patents, China's latest patents, Technical Efficacy Thesaurus, Application Domain, Technology Topic.
© 2024 PatSnap. All rights reserved.Legal|Privacy policy|Modern Slavery Act Transparency Statement|Sitemap