Radar processor system and method
a radar and processor technology, applied in the field of radar processing methods and systems, can solve problems such as non-homogeneous clutter, jamming, dense target clusters, etc., and achieve the effects of improving the performance and efficiency of the algorithm, and improving the computational speed of fracta
- Summary
- Abstract
- Description
- Claims
- Application Information
AI Technical Summary
Benefits of technology
Problems solved by technology
Method used
Image
Examples
Embodiment Construction
[0039] Referring now to FIG. 1, a radar system 100 includes an N-element uniform linear array 102 resulting in N Radio Frequency (RF) input channels. Each of the N array elements has M time delayed inputs 104 which are combined via adaptive linear weighting to form outputs 106 such that an output performance measure (such as signal-to-noise (S / N) power ratio) is optimized. The adaptive linear weighting is determined by a STAP processor 105. A combiner 108 accumulates the weighted outputs to form the final output power residue.
[0040] Assume that for each of these RF channels, the radar front end carries out amplification, filtering, reduction to baseband, and analog-to-digital (A / D) conversion. The output of each A / D is a data stream of in-phase and quadrature phase (I, Q) output pairs. The I and Q components represent the real and imaginary parts, respectively, of the complex valued data stream. The radar waveform is assumed to be a burst of M identical pulses with pulse repetition...
PUM
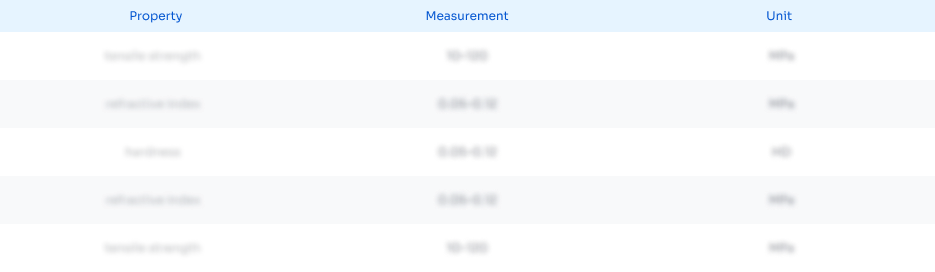
Abstract
Description
Claims
Application Information
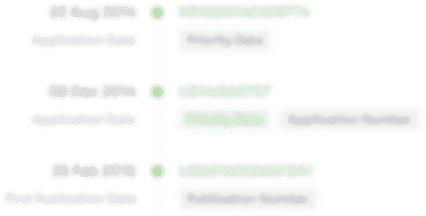
- R&D Engineer
- R&D Manager
- IP Professional
- Industry Leading Data Capabilities
- Powerful AI technology
- Patent DNA Extraction
Browse by: Latest US Patents, China's latest patents, Technical Efficacy Thesaurus, Application Domain, Technology Topic.
© 2024 PatSnap. All rights reserved.Legal|Privacy policy|Modern Slavery Act Transparency Statement|Sitemap