Tricholoma matsutake fast nondestructive testing system and method based on convolutional neural network
A convolutional neural network and non-destructive testing technology, applied in neural learning methods, biological neural network models, neural architectures, etc., can solve problems such as low classification accuracy, misleading consumption, and loss of matsutake water, reducing the amount of calculation and storage requirements , ensure accuracy and completeness, and improve the effectiveness of valid information
- Summary
- Abstract
- Description
- Claims
- Application Information
AI Technical Summary
Problems solved by technology
Method used
Image
Examples
Embodiment Construction
[0054] The present invention will be further described below in conjunction with accompanying drawing.
[0055] The present invention combines deep learning network and near-infrared spectroscopy technology, and proposes a rapid non-destructive detection method for matsutake and other rare edible fungi according to domestic consumption of residents and the status quo of national import and export trade. figure 1 It is an overall architecture diagram of the present invention, which shows the relationship between the deep learning model 160, the control terminal 190, the handheld terminal 170, the consumer 180 and the supervision department 210. After the deep learning model 160 detects the matsutake index results, it will detect The results are stored in the cloud server at the control end, and the consumer 180 accesses the cloud server through the APP installed on the handheld terminal 170 to obtain the test results; meanwhile, the supervisory department 210 can also access the...
PUM
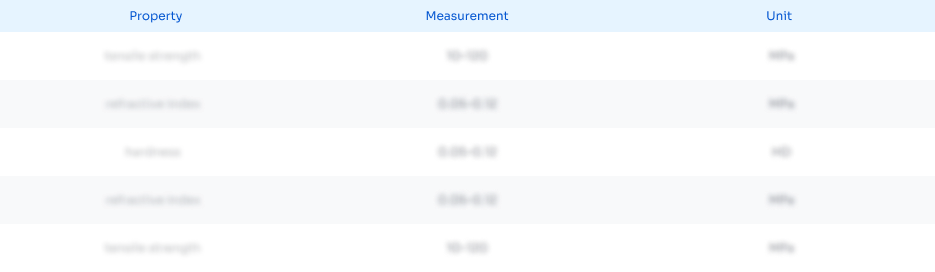
Abstract
Description
Claims
Application Information
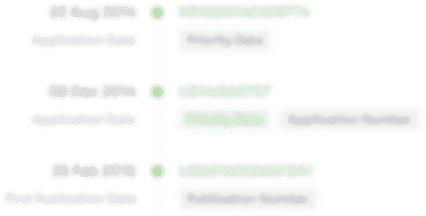
- R&D Engineer
- R&D Manager
- IP Professional
- Industry Leading Data Capabilities
- Powerful AI technology
- Patent DNA Extraction
Browse by: Latest US Patents, China's latest patents, Technical Efficacy Thesaurus, Application Domain, Technology Topic, Popular Technical Reports.
© 2024 PatSnap. All rights reserved.Legal|Privacy policy|Modern Slavery Act Transparency Statement|Sitemap|About US| Contact US: help@patsnap.com