A real-time monocular video depth estimation method
A technology of depth estimation and video, applied in neural learning methods, calculations, computer components, etc., can solve problems such as limiting the practicality of depth estimation, and achieve the effect of promoting practicality, less model parameters, and promoting development
- Summary
- Abstract
- Description
- Claims
- Application Information
AI Technical Summary
Problems solved by technology
Method used
Image
Examples
Embodiment Construction
[0041] The present invention will now be further described in conjunction with the embodiments and accompanying drawings:
[0042] The technical scheme of the present invention is to combine a two-dimensional convolutional neural network (2D-CNN) and a convolutional long-short-term memory (CLSTM) network to construct a pair of A model for real-time deep depth estimation from monocular video data. At the same time, a generative adversarial network (GAN) is used to constrain the estimated results to satisfy temporal consistency.
[0043] The specific measures of this technical solution are as follows:
[0044] Step 1: Data preprocessing. Data preprocessing includes RGB video normalization, depth map normalization and sample extraction.
[0045] Step 2: Divide the training set and the validation set. A small number of samples are extracted as the validation set, and all the remaining samples are used as the training set.
[0046] Step 3: Build the network model. In the pres...
PUM
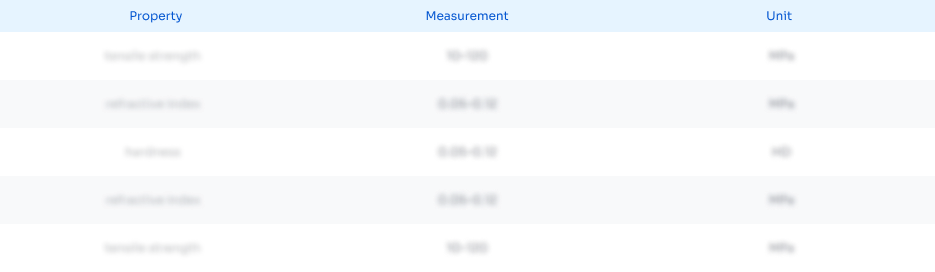
Abstract
Description
Claims
Application Information
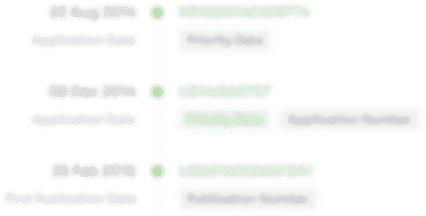
- R&D
- Intellectual Property
- Life Sciences
- Materials
- Tech Scout
- Unparalleled Data Quality
- Higher Quality Content
- 60% Fewer Hallucinations
Browse by: Latest US Patents, China's latest patents, Technical Efficacy Thesaurus, Application Domain, Technology Topic, Popular Technical Reports.
© 2025 PatSnap. All rights reserved.Legal|Privacy policy|Modern Slavery Act Transparency Statement|Sitemap|About US| Contact US: help@patsnap.com