Deep learning-based power control method for user-centric network
A deep learning and power control technology, applied in the field of interference management, which can solve the problems of gradient disappearance, reduced fitting performance, and unsatisfactory fitting effect.
- Summary
- Abstract
- Description
- Claims
- Application Information
AI Technical Summary
Problems solved by technology
Method used
Image
Examples
Embodiment Construction
[0058] The implementation cases of the present invention are described in detail in conjunction with the accompanying drawings.
[0059] attached figure 1 It is a schematic diagram of user-centric power control mechanism and base station scheduling correlation scenario. The present invention is mainly applied in a homogeneous network where dense small cells are deployed, that is, a large number of small cells are deployed in an LTE network, and base stations and users are equipped with multiple antennas. Usually, the distribution of multiple small stations is random. All BSs and users are randomly placed in an ultra-dense square area of 1000X1000 meters, following an independent Poisson point process distribution. A channel model consisting of two parts is adopted: 1) The path loss model is PL i,j =146.1+37.6log 10 d i,j (dB), where d i,j (in km) is user i and BS j 2) using flat Rayleigh fading, where each element of the channel is an independent and identically dist...
PUM
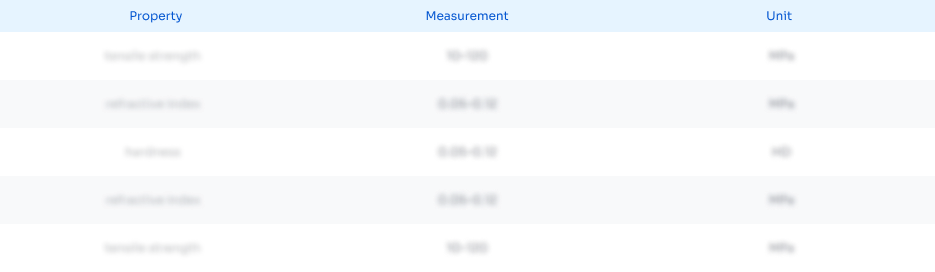
Abstract
Description
Claims
Application Information
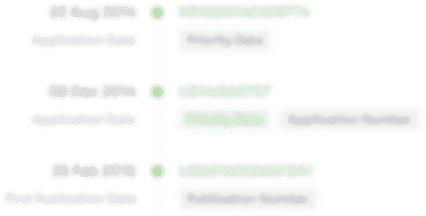
- R&D Engineer
- R&D Manager
- IP Professional
- Industry Leading Data Capabilities
- Powerful AI technology
- Patent DNA Extraction
Browse by: Latest US Patents, China's latest patents, Technical Efficacy Thesaurus, Application Domain, Technology Topic, Popular Technical Reports.
© 2024 PatSnap. All rights reserved.Legal|Privacy policy|Modern Slavery Act Transparency Statement|Sitemap|About US| Contact US: help@patsnap.com