Method and system for training heart motion field estimation model and method and system for heart motion field estimation
An estimation model and motion field technology, applied in the field of image processing, can solve the problem of low accuracy of cardiac motion estimation, and achieve the effect of overcoming the low accuracy of cardiac motion estimation
- Summary
- Abstract
- Description
- Claims
- Application Information
AI Technical Summary
Problems solved by technology
Method used
Image
Examples
Embodiment 1
[0067] A method for training a cardiac motion field estimation model provided by an embodiment of the present invention, such as figure 1 shown, including the following steps:
[0068] Step S1: Construct a semi-autoencoder network with preset network parameters.
[0069] The semi-autoencoder network includes an encoding layer, a deformation field calculation layer, and a spatial deformation layer, wherein the encoding layer includes three layers of convolutional networks with different resolutions, wherein;
[0070] The first layer is a convolution layer, the convolution kernel size of the convolution layer is 3×3, the number of convolution kernels is 16, and the two input images are concatenated as the input data of the convolution layer; the second layer It is composed of 4 convolutional layers cascaded. The size of the convolutional kernel of each convolutional layer is 3×3, and the number of convolutional kernels is 32. It is followed by a downsampling operation. The size...
Embodiment 2
[0116] An embodiment of the present invention provides a method for cardiac motion field estimation, such as Figure 4 shown, including the following steps:
[0117] Step S21: Obtain images of the end-diastole and end-systole to be estimated.
[0118] In the embodiment of the present invention, the cardiac image may be acquired by an image device, for example, a short-axis cardiac image may be obtained by a Cine Magnetic Resonance (Cine Magnetic Resonance, Cine-MR) device.
[0119] Step S22: Input the images of the end-diastole and end-systole to be estimated into the cardiac motion field estimation model obtained according to the method for training the cardiac motion field estimation model described in Example 1, and obtain the distribution parameters of invisible variable parameters.
[0120] Step S23: Sampling the distribution of the cloaking variable parameters to obtain the cloaking variable parameter values, constructing a dense deformation field using the radial basis...
Embodiment 3
[0127] An embodiment of the present invention provides a computer device, such as Figure 6 As shown, it includes: at least one processor 401 , such as a CPU (Central Processing Unit, central processing unit), at least one communication interface 403 , memory 404 , and at least one communication bus 402 . Wherein, the communication bus 402 is used to realize connection and communication between these components. Wherein, the communication interface 403 may include a display screen (Display) and a keyboard (Keyboard), and the optional communication interface 403 may also include a standard wired interface and a wireless interface. The memory 404 may be a high-speed RAM memory (Ramdom Access Memory, volatile random access memory), or a non-volatile memory (non-volatile memory), such as at least one disk memory. Optionally, the memory 404 may also be at least one storage device located away from the aforementioned processor 401 . The processor 401 may execute the method for tra...
PUM
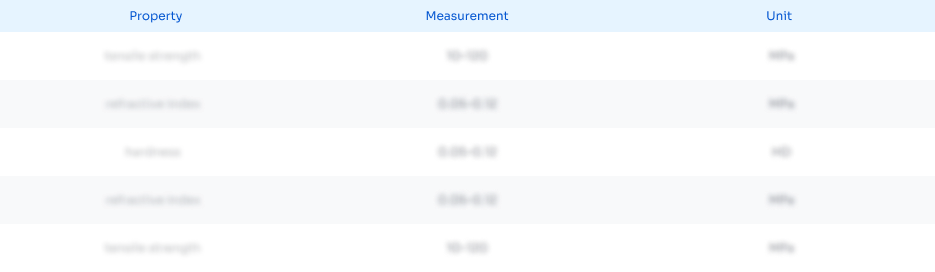
Abstract
Description
Claims
Application Information
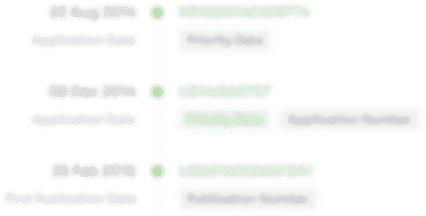
- R&D Engineer
- R&D Manager
- IP Professional
- Industry Leading Data Capabilities
- Powerful AI technology
- Patent DNA Extraction
Browse by: Latest US Patents, China's latest patents, Technical Efficacy Thesaurus, Application Domain, Technology Topic, Popular Technical Reports.
© 2024 PatSnap. All rights reserved.Legal|Privacy policy|Modern Slavery Act Transparency Statement|Sitemap|About US| Contact US: help@patsnap.com