Graph convolutional network biomedical information extraction method based on multi-head attention mechanism
A biomedical and convolutional network technology, applied in the field of biomedical information extraction, can solve problems such as performance degradation, difficulty in meeting the requirements of extraction tasks, and difficulty in detecting cross-sentence entities, so as to improve performance and reduce the impact of noisy data Effect
- Summary
- Abstract
- Description
- Claims
- Application Information
AI Technical Summary
Problems solved by technology
Method used
Image
Examples
Embodiment 1
[0088] A biomedical information extraction method using a graph convolutional network based on a multi-head attention mechanism, which is applied to the following scenarios:
[0089] Dataset: Two public biomedical datasets are used: CDR corpus and Chemprot corpus. At the same time, a cross-institutional dataset of cancer pathology reports is constructed. The characteristics are as follows, CDR corpus: whether there is a relationship between chemical substances and diseases.
[0090] Chemprot corpus: whether there is a relationship between chemicals and proteins;
[0091] Cancer pathology report: including cancer type, tumor resection location, largest tumor diameter, histological subtype, histological grade, TNM stage and lymph node metastasis.
[0092] Specific steps are as follows:
[0093] 1. Establish a graph convolutional network model based on the multi-head attention mechanism for the text data information of the dataset
[0094] 1.1 Use python to implement the initia...
PUM
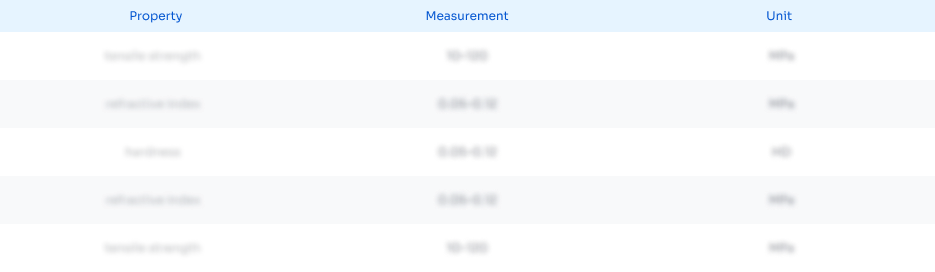
Abstract
Description
Claims
Application Information
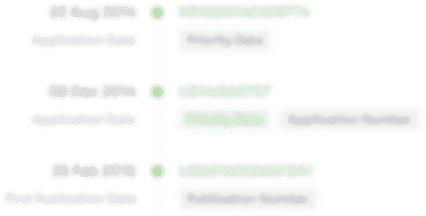
- R&D Engineer
- R&D Manager
- IP Professional
- Industry Leading Data Capabilities
- Powerful AI technology
- Patent DNA Extraction
Browse by: Latest US Patents, China's latest patents, Technical Efficacy Thesaurus, Application Domain, Technology Topic, Popular Technical Reports.
© 2024 PatSnap. All rights reserved.Legal|Privacy policy|Modern Slavery Act Transparency Statement|Sitemap|About US| Contact US: help@patsnap.com