Missile-borne image deblurring method based on generative adversarial network
A technology for deblurring and image, which is applied in biological neural network model, image enhancement, image analysis, etc. It can solve problems such as jitter, difficulty in accurate identification and blurring of missile-borne image targets, and improve detection accuracy and efficiency, and improve PSNR and SSIM indicators, the effect of eliminating ambiguous information
- Summary
- Abstract
- Description
- Claims
- Application Information
AI Technical Summary
Problems solved by technology
Method used
Image
Examples
Embodiment
[0092] Embodiment: Deblurring the missile-borne image.
[0093] Such as figure 1 , 2 As shown, a method for deblurring missile-borne images based on an adversarial generation network disclosed in this embodiment includes the following steps:
[0094] Step 1: Make a training set of fuzzy data of missile-borne images.
[0095] Due to the difficulty in obtaining real strike scene data, according to the imaging characteristics of the missile-borne image, the scene of the field of view of the seeker is simulated by the scene generation server as the main source of image data, as shown in the attached image 3 As shown, the visual display screen generates the image visual of the seeker, and the development board collects the missile-borne image in real time through the camera, and the collected result is displayed on the monitor in real time, and this data is used as the main sample for training.
[0096] The specific implementation method of step 1 is:
[0097] Step 1.1: Manual...
Embodiment 2
[0154] Embodiment 2: Indirectly prove the effectiveness of the deblurring algorithm from the accuracy of target detection.
[0155] Step 1: Make a training set of missile-borne image fuzzy data.
[0156] Using the hardware-in-the-loop simulation test platform, 1000 pairs of missile-borne image fuzzy data sets with targets to be hit are produced, of which 900 pairs are used as training sets and 100 pairs are used as test sets.
[0157] Step 2: Establish a forward propagation model against the network.
[0158] The overall structure of the missile-borne image deblurring model is as follows: figure 2 As shown in (a), first input the blurred image into the generator and output an image to be judged, then take a corresponding clear image from the clear image set, and put the generator output and clear image into an image pair into the discriminator , to judge which of the two images is a clear image and which is a forged image by the generator. The goal of the generator is to g...
PUM
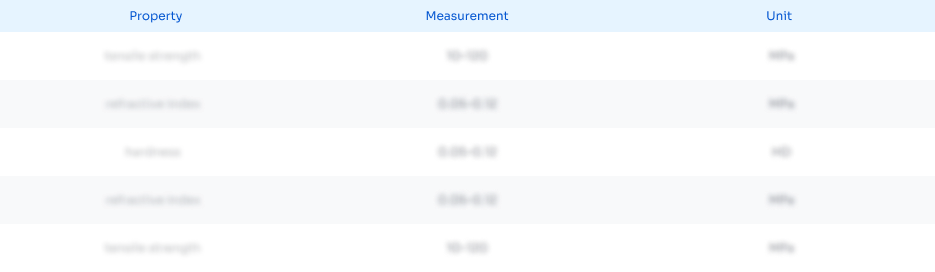
Abstract
Description
Claims
Application Information
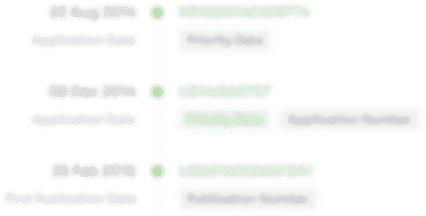
- R&D Engineer
- R&D Manager
- IP Professional
- Industry Leading Data Capabilities
- Powerful AI technology
- Patent DNA Extraction
Browse by: Latest US Patents, China's latest patents, Technical Efficacy Thesaurus, Application Domain, Technology Topic, Popular Technical Reports.
© 2024 PatSnap. All rights reserved.Legal|Privacy policy|Modern Slavery Act Transparency Statement|Sitemap|About US| Contact US: help@patsnap.com