Near-surface air temperature inversion method based on temperature, emissivity and deep learning
A deep learning and air temperature technology, applied in neural learning methods, complex mathematical operations, biological neural network models, etc., can solve problems such as low universality, and achieve the effect of increasing universality and high inversion accuracy
- Summary
- Abstract
- Description
- Claims
- Application Information
AI Technical Summary
Problems solved by technology
Method used
Image
Examples
Embodiment 1
[0029] according to figure 1 As shown, this embodiment proposes a near-surface air temperature inversion method based on temperature and emissivity and deep learning, including the following steps:
[0030] Step 1. Establish the heat radiation transfer equation
[0031] Determine the research area, obtain the satellite remote sensing sensor data and ERA5-Land data in the research area, establish a model data set based on the obtained satellite remote sensing sensor data and ERA5-Land data, and introduce auxiliary data into the model data set, and then obtain the data from the satellite remote sensing The thermal radiation transfer equation is established from the perspective of the sensor data, where the ERA5-Land data is generated by the European Center for Medium-Range Weather Forecast by reconstructing the ERA5 climate in the land part of the analysis data set. Decades of land variable reanalysis data are available at high resolution;
[0032] Step 2: Build an expert know...
Embodiment 2
[0065] This embodiment proposes a near-surface air temperature inversion method based on temperature and emissivity and deep learning, including the following steps:
[0066] Step 1. Establish the heat radiation transfer equation
[0067] Determine the research area, obtain the satellite remote sensing sensor data and ERA5-Land data in the research area, establish a model data set based on the obtained satellite remote sensing sensor data and ERA5-Land data, and introduce auxiliary data into the model data set, and then obtain the data from the satellite remote sensing The angle of the sensor data establishes the heat radiation transfer equation;
[0068] Step 2: Build an expert knowledge base
[0069] Based on the heat radiation transfer equation established in step 1, combined with the existing prior knowledge, an expert knowledge base is constructed, and then physical and logical reasoning is carried out through the constructed expert knowledge base to derive the parameter...
PUM
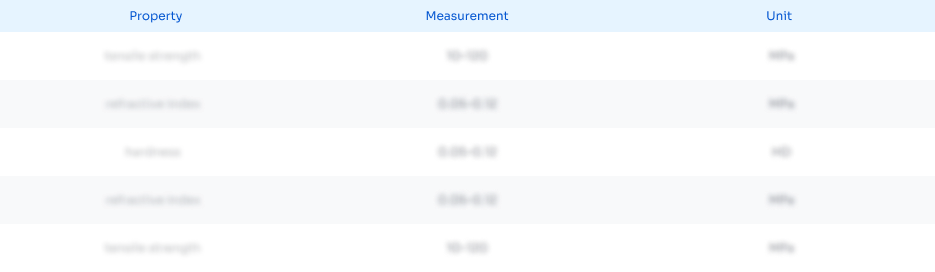
Abstract
Description
Claims
Application Information
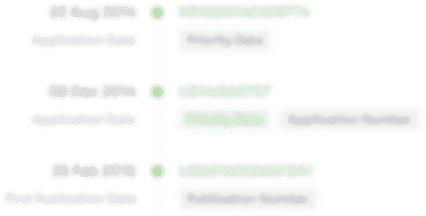
- R&D Engineer
- R&D Manager
- IP Professional
- Industry Leading Data Capabilities
- Powerful AI technology
- Patent DNA Extraction
Browse by: Latest US Patents, China's latest patents, Technical Efficacy Thesaurus, Application Domain, Technology Topic.
© 2024 PatSnap. All rights reserved.Legal|Privacy policy|Modern Slavery Act Transparency Statement|Sitemap