FIB-SEM super-resolution algorithm based on generative adversarial network
A FIB-SEM and super-resolution technology, which is applied in the field of FIB-SEM super-resolution algorithm, can solve problems such as artifacts, image dotted lines, singleness, etc., and achieve the effect of obvious texture, good effect, and simple and intuitive algorithm
- Summary
- Abstract
- Description
- Claims
- Application Information
AI Technical Summary
Problems solved by technology
Method used
Image
Examples
Embodiment 1
[0047] The discriminator module of the present invention is mainly used to convert a low-resolution image into a high-resolution image with two different feature vectors through a sampling interpolation algorithm, and use the vector feature as a criterion to judge the authenticity of the image resolution. This module is mainly divided into 6 steps. The low-resolution and high-resolution features are used as the division criteria, and the low-resolution and corresponding real high-resolution images are used as a set of training sets. According to the training set: test set: validation set = 7:2:1, and divide the image into two groups of high-resolution and low-resolution image data, calculate and optimize the training loss of the obtained image, and use the sigmoid activation function to activate the feature vector and send it to the discrimination module. Different index values are used to judge the authenticity of the image resolution.
Embodiment 2
[0049] The generator module of the present invention is mainly used for converting a low-resolution image into a predetermined high-resolution image. This module is completed in 3 steps.
[0050] Specific steps are as follows:
[0051] Step1: Generate high-resolution images: Read in the low-resolution image data input by the discriminator, process the image data to generate 3*3 convolutional neural network + BN + PRelu modules and 8 residual modules, each residual The module consists of a 3*3 convolution kernel conv+BN+Relu+conv module, and assigns different channel numbers to it, and then uses a 3*3 convolutional neural network+BN+Relu module to control the output channel to 64 , with a step size of 1. After that, the shallow features obtained by the first convolution are combined with the corresponding convolution semantic features here, and then the super-resolution magnification is performed by convolution + PixelShuffle + PreLU, and the magnification size is N*W, N* H....
PUM
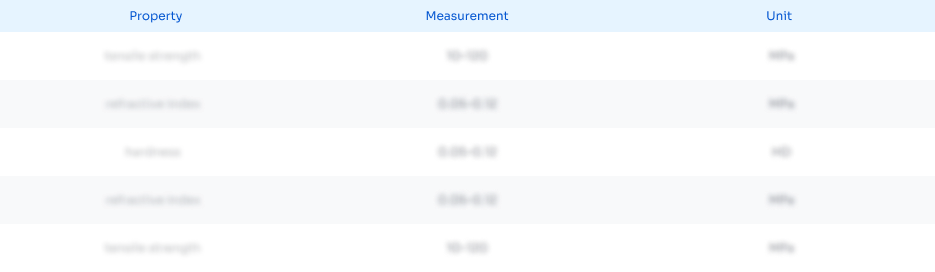
Abstract
Description
Claims
Application Information
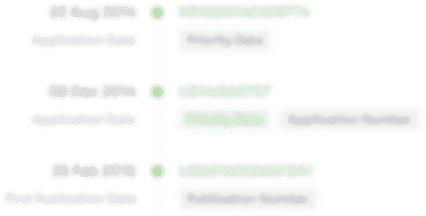
- Generate Ideas
- Intellectual Property
- Life Sciences
- Materials
- Tech Scout
- Unparalleled Data Quality
- Higher Quality Content
- 60% Fewer Hallucinations
Browse by: Latest US Patents, China's latest patents, Technical Efficacy Thesaurus, Application Domain, Technology Topic, Popular Technical Reports.
© 2025 PatSnap. All rights reserved.Legal|Privacy policy|Modern Slavery Act Transparency Statement|Sitemap|About US| Contact US: help@patsnap.com