Intelligent prediction method and system for NSCLC lymph node metastasis risk
A technology for lymph node metastasis and intelligent prediction, applied in neural learning methods, diagnostic recording/measurement, instruments, etc., can solve problems such as lack of technology, cost of time, reagents, consumables, etc., and achieve the effect of shortening operation time and reducing the probability of tumor recurrence.
- Summary
- Abstract
- Description
- Claims
- Application Information
AI Technical Summary
Problems solved by technology
Method used
Image
Examples
Embodiment 1
[0053] participate Figure 1 to Figure 3 As shown, a CNN-based intelligent prediction method for the risk of lymph node metastasis in NSCLC includes the following steps:
[0054] S1: Obtain the full-field digital image (WSI) of the conventional pathological H&E-stained slides of the surgically resected T1-2 lung cancer mass, and additionally obtain the full-field digital image (WSI) of the pathological H&E-stained slides of normal lung tissue; all images Screened and confirmed by a pathologist;
[0055] S2: The pathologist marks the normal tissue area in the H&E full-field digital slice of lung cancer; the pathologist selects the full-field digital slice of normal lung tissue, and the image does not contain tumor tissue;
[0056] S3: Cut the color block on the acquired image;
[0057] S4: Divide the color patch images into a training set and a validation set according to the cases they belong to. Each set contains 1:1 tumor and non-tumor color patches. The constructed convol...
Embodiment 2
[0078] Another object of the present invention is to provide an intelligent prediction system for NSCLC lymph node metastasis risk based on CNN, including: an input terminal module, a single case processing program module, a learning optimization program module, and a report output terminal module;
[0079] The input terminal module includes performing quality control on the input full-field digital image of lung cancer, and is used to remove the normal tissue color patches next to the cancer, remove the high background color patches, and retain and reorganize the tumor tissue color patches to achieve the preprocessing effect;
[0080] The single case processing program module includes using the previously generated convolutional neural network model to analyze the image to obtain the characteristics of each characteristic color block, and finally to determine the risk;
[0081] The learning optimization program module is used to batch receive full-field digital images of lung ...
Embodiment 3
[0084] data collection:
[0085] The pathological diagnosis of lung non-small cell carcinoma and T staging ≤ T2 in the Department of Pathology, The First Affiliated Hospital of Nanchang University from January 2019 to November 2021 were collected, and all H&E stained sections were screened; and relevant clinical data of the patients were obtained. Pathological special data.
[0086] Quality control:
[0087] Severely decolorized or stained glass slides were excluded from H&E stained slides, and infection, fibrosis, and H&E stained slides affecting normal lung tissue were excluded from H&E stained slides of normal lung tissue; the visual quality of tumor tissue was excluded due to technical artifacts. Such as air bubbles, pen marks, water droplets, dust, air inclusions, hair, etc. on glass slides; all images of full-field digital slices were evaluated by three pathology experts, and images were selected 1:1 into tumor tissue and Non-tumor tissue; images with inconsistent diag...
PUM
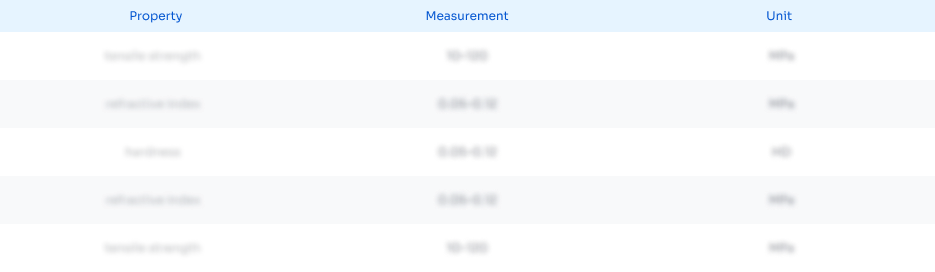
Abstract
Description
Claims
Application Information
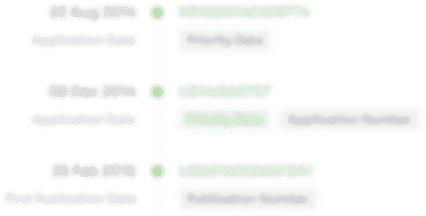
- R&D Engineer
- R&D Manager
- IP Professional
- Industry Leading Data Capabilities
- Powerful AI technology
- Patent DNA Extraction
Browse by: Latest US Patents, China's latest patents, Technical Efficacy Thesaurus, Application Domain, Technology Topic, Popular Technical Reports.
© 2024 PatSnap. All rights reserved.Legal|Privacy policy|Modern Slavery Act Transparency Statement|Sitemap|About US| Contact US: help@patsnap.com