Prostate image segmentation method
An image segmentation and prostate technology, applied in the field of medical images, can solve the problems of indistinguishable borders, dependence, long algorithm time consumption, etc., and achieve the effect of fast running speed and good robustness
- Summary
- Abstract
- Description
- Claims
- Application Information
AI Technical Summary
Problems solved by technology
Method used
Image
Examples
Embodiment 1
[0063] Embodiment 1 An image segmentation method based on a traditional convolutional neural network (CNN) consists of a convolutional layer, a pooling layer, a fully connected layer and a softmax classifier layer. as attached figure 2 , after the image undergoes a series of convolution, pooling and full connection, the output feature vector can accurately identify the image category.
[0064] Layer l convolution feature map h l The calculation method is:
[0065]
[0066] In the formula, Mx and My represent the length and width of the convolution filter M, respectively, and w jk is the weight learned in the convolution kernel, h l-1 Indicates the input of the convolutional layer l, bl Represents the bias of the l-th layer filter, f(·) is the activation function. The popular deep neural network mostly uses the ReLu activation function instead of the traditional Sigmoid function to accelerate network convergence. Its mathematical expression is:
[0067] f(x)=max(0,x) ...
Embodiment 2
[0071] Embodiment 2 The image segmentation method based on the fully convolutional neural network (FCN) is based on the CNN classification network, as attached image 3 , convert the fully connected layer into a convolutional layer to preserve the spatial two-dimensional information, then deconvolve the convolutional two-dimensional feature map to restore the original image size, and finally obtain each pixel category by pixel-by-pixel classification, so as to achieve Image segmentation purpose.
[0072] The present invention can adopt the idea of fuzzy sets based on degree of membership to classify, adopt
[0073] e is the natural logarithm, and a and c are parameters. π-type functions can be defined by sigmoid functions.
[0074] From the perspective of pixel classification, the standard S-shaped function conforms to the transition process of the prostate image edge, so the invention point of this embodiment is to use the S-shaped function as the basic transformation f...
Embodiment 3
[0091] Embodiment 3 The present invention is based on the two-dimensional image segmentation method of the improved full convolutional neural network (FCN), as attached Figure 4 , follow the steps below:
[0092] S1. Obtain the training samples of the prostate region and mark them;
[0093] S11. Use a 1.5T magnetic resonance system and an 8-channel phased array to perform spin echo single-shot EPI imaging on the training prostate. The imaging parameters are: TR 4800-5000ms, TE 102ms, slice thickness 3.0mm, slice distance 0.5 mm, echo chain length 24, phase 256, frequency 288, NEX 4.0, bandwidth 31.255kHz, pixel 512×512;
[0094] S12. Workers use MITK software to manually segment the image.
[0095] S2. Perform preprocessing on the training prostate area to obtain a preprocessing result;
[0096] S21. Calculate the average intensity value and standard deviation of all training images;
[0097] S22. Performed a normalization operation, including subtracting the mean and div...
PUM
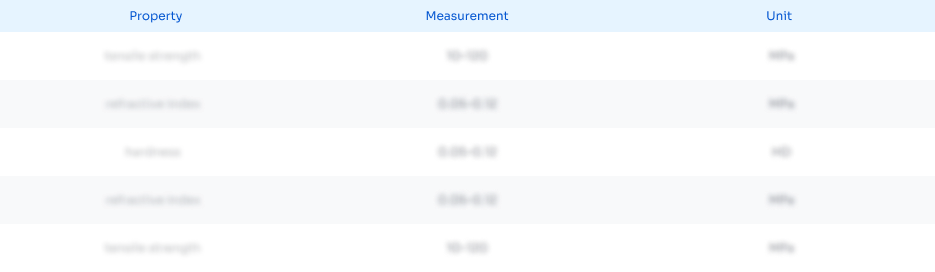
Abstract
Description
Claims
Application Information
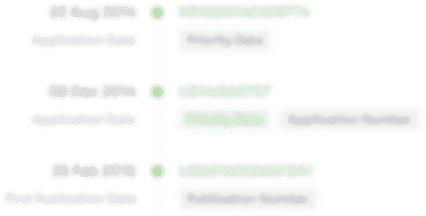
- R&D Engineer
- R&D Manager
- IP Professional
- Industry Leading Data Capabilities
- Powerful AI technology
- Patent DNA Extraction
Browse by: Latest US Patents, China's latest patents, Technical Efficacy Thesaurus, Application Domain, Technology Topic.
© 2024 PatSnap. All rights reserved.Legal|Privacy policy|Modern Slavery Act Transparency Statement|Sitemap