Rolling bearing prediction method and system based on local binary pattern and deep belief network
A local binary mode, deep belief network technology, applied in the fields of mechanical equipment health detection and image processing, can solve problems such as high computational consumption, limited classification accuracy, poor combination of bearing diagnosis methods and image processing methods, etc.
- Summary
- Abstract
- Description
- Claims
- Application Information
AI Technical Summary
Problems solved by technology
Method used
Image
Examples
Embodiment
[0170] 1. Raw data preparation
[0171]This embodiment takes the bearing data of Western Reserve University as an example to illustrate the implementation method of rolling bearing fault diagnosis based on local binary model and deep belief network.
[0172] The rolling bearing test platform includes a 2-horsepower motor (left side) (1hp=746w), a torque sensor (middle), a dynamometer and electronic control equipment. Use electric discharge machining technology to arrange single-point faults on the inner ring, outer ring and rolling elements of rolling bearings, and the fault diameters are 0.007, 0.014, and 0.021 inches respectively. Bearings used are four SKF bearings. The test bench includes the drive end bearing and the fan end bearing, and the acceleration sensor is installed on the motor housing and the 12 o'clock position of the drive end and fan end respectively. The vibration signal is collected by a general-purpose 6-channel DAT recorder. The sampling frequency of th...
PUM
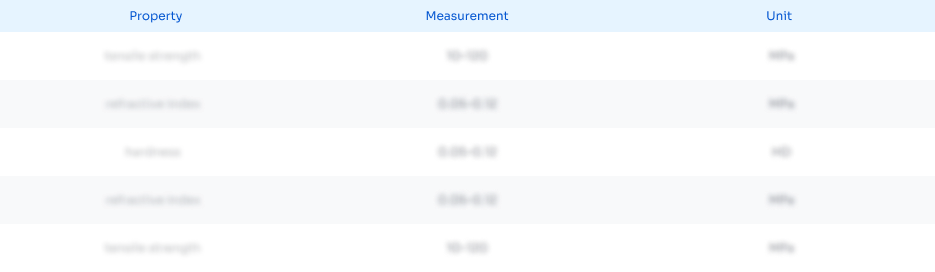
Abstract
Description
Claims
Application Information
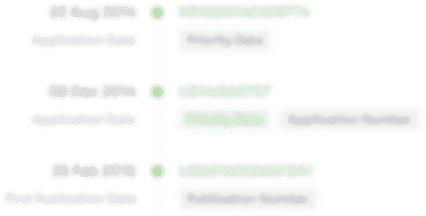
- R&D Engineer
- R&D Manager
- IP Professional
- Industry Leading Data Capabilities
- Powerful AI technology
- Patent DNA Extraction
Browse by: Latest US Patents, China's latest patents, Technical Efficacy Thesaurus, Application Domain, Technology Topic, Popular Technical Reports.
© 2024 PatSnap. All rights reserved.Legal|Privacy policy|Modern Slavery Act Transparency Statement|Sitemap|About US| Contact US: help@patsnap.com