Scheduling method and device based on deep learning node calculation and storage medium
A scheduling method and deep learning technology, applied in the field of deep learning, can solve the problems of hardware idleness, unfavorable acceleration, and only applicable data flow chips, etc., and achieve the effect of asynchronous calculation process
- Summary
- Abstract
- Description
- Claims
- Application Information
AI Technical Summary
Problems solved by technology
Method used
Image
Examples
Embodiment 1
[0014] Such as figure 1 As shown, an embodiment of the present invention provides a scheduling method based on deep learning node calculation, and the scheduling method based on deep learning node calculation includes steps S110 to S140.
[0015] In step S110, the nodes to be calculated of the preset neural network calculation graph are acquired.
[0016] In this embodiment, the neural network is a complex network system formed by extensive interconnection of a large number of simple processing units (also called neurons), which reflects many basic features of human brain functions and is a highly complex network system. nonlinear dynamic learning system. In the calculation graph of the actual calculation process of the neural network, the simplest calculation unit is called a calculation node, and each calculation node that has not been calculated is a node to be calculated. Obtain the nodes to be calculated in the preset neural network calculation graph, that is, insert th...
Embodiment 2
[0025] Such as figure 2 As shown, Embodiment 2 of the present invention is further optimized on the basis of Embodiment 1 of the present invention. Embodiment 2 of the present invention provides a scheduling method based on deep learning node calculation. The scheduling method based on deep learning node calculation includes Step S100 to step S422.
[0026] In step S100, the nodes to be calculated of the preset neural network calculation graph are obtained.
[0027] In this embodiment, the neural network is a complex network system formed by extensive interconnection of a large number of simple processing units (also called neurons), which reflects many basic features of human brain functions and is a highly complex network system. nonlinear dynamic learning system. In the calculation graph of the actual calculation process of the neural network, the simplest calculation unit is called a calculation node, and each calculation node that has not been calculated is a node to b...
Embodiment 3
[0052] image 3 It is a schematic structural diagram of a scheduling device based on deep learning node computing provided by Embodiment 3 of the present invention. image 3 A block diagram of an exemplary deep learning node computing-based scheduling device 12 suitable for implementing embodiments of the present invention is shown. image 3 The displayed scheduling device 12 based on deep learning node calculation is only an example, and should not impose any limitation on the functions and scope of use of the embodiments of the present invention.
[0053] Such as image 3 As shown, the scheduling device 12 based on deep learning node computing is represented in the form of a general-purpose computing device. Components of the scheduling device 12 based on deep learning node calculations may include, but are not limited to: at least one processor or processing unit 16, a storage device 28, and a bus 18 connecting different system components (including the storage device 28 ...
PUM
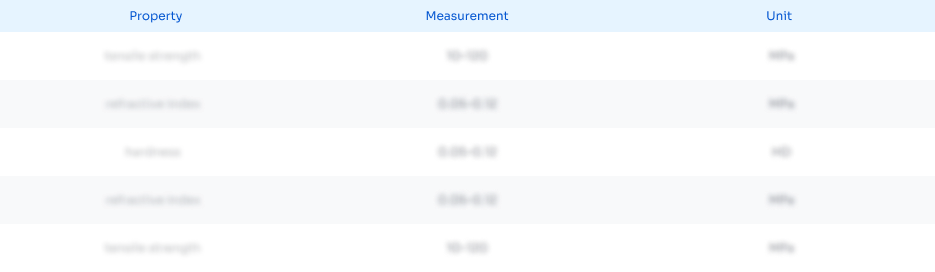
Abstract
Description
Claims
Application Information
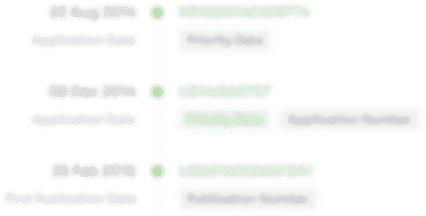
- R&D
- Intellectual Property
- Life Sciences
- Materials
- Tech Scout
- Unparalleled Data Quality
- Higher Quality Content
- 60% Fewer Hallucinations
Browse by: Latest US Patents, China's latest patents, Technical Efficacy Thesaurus, Application Domain, Technology Topic, Popular Technical Reports.
© 2025 PatSnap. All rights reserved.Legal|Privacy policy|Modern Slavery Act Transparency Statement|Sitemap|About US| Contact US: help@patsnap.com