Multi-beam seabed sedimentary layer type estimation method and system based on transfer learning
A technology of seabed deposition and migration learning, which is applied in the fields of seabed detection and hydroacoustic physics, can solve the problems of reduced model generalization performance and reduced prediction accuracy, and achieves the effect of satisfying real-time processing, short time and good training effect.
- Summary
- Abstract
- Description
- Claims
- Application Information
AI Technical Summary
Problems solved by technology
Method used
Image
Examples
Embodiment 1
[0078] Multi-beam data acquisition experiments have been carried out in a test sea area for two years. The water depth of the test sea area is 30-60m, figure 1 The route trajectory and seabed sediment type distribution of the two test navigation measurements are given, according to figure 1 As shown, it can be seen that the test route of the dotted line E1 to E2 is parallel to the test route 2 of the dotted line A to B, and the types of the covered seabed sediments (sea bottom) are basically the same, and the types of the seabed sediments are clay powder. Sand, sandy silt and silt. The same NORBIT WBMS Bathy 200 multi-beam bathymetric sonar system was used in the two tests, and the QINSY software was used to collect seabed backscatter data; in the two tests, the parameter settings of the sonar system used were the same. In the multi-beam data processing, in order to avoid the random fluctuation of the data, the data of the two experiments were overlapped and averaged by mult...
Embodiment 2
[0093] The present invention also provides a multi-beam seabed sediment type estimation system based on migration learning, the system specifically includes:
[0094] The migration module is used to use part of the data in the multi-beam echo intensity data on the survey line of the target sea area as migration data, and input it into the pre-trained deep convolutional neural network model, and the part of the deep convolutional neural network model Fine-tuning network node parameters to obtain a revised neural network model; and
[0095] The estimation module is used to input the real-time received multi-beam echo intensity data on the survey line of the target sea area into the corrected neural network model, and output the multi-beam seabed sediment layer type.
Embodiment 3
[0097] Embodiment 3 of the present invention may further provide a computer device, including: at least one processor, a memory, at least one network interface, and a user interface. The individual components in the device are coupled together via a bus system. It can be understood that the bus system is used to realize the connection communication between these components. In addition to the data bus, the bus system also includes a power bus, a control bus and a status signal bus.
[0098] Wherein, the user interface may include a display, a keyboard, or a pointing device (for example, a mouse, a trackball (trackball), a touch panel, or a touch screen, and the like.
[0099] It can be understood that the memory in the disclosed embodiments of the present application may be a volatile memory or a non-volatile memory, or may include both volatile and non-volatile memory. Wherein, the non-volatile memory may be a read-only memory (Read-Only Memory, ROM), a programmable read-on...
PUM
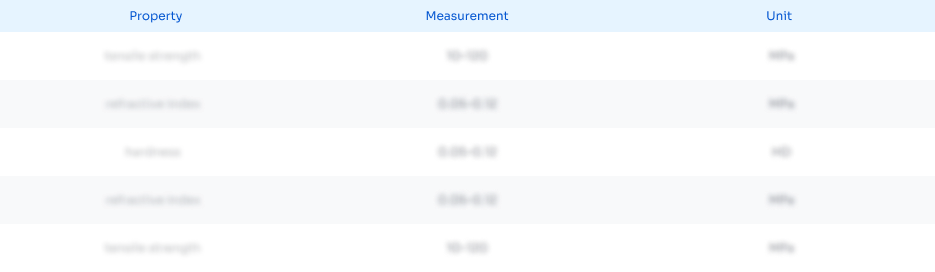
Abstract
Description
Claims
Application Information
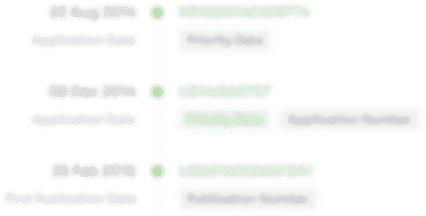
- R&D Engineer
- R&D Manager
- IP Professional
- Industry Leading Data Capabilities
- Powerful AI technology
- Patent DNA Extraction
Browse by: Latest US Patents, China's latest patents, Technical Efficacy Thesaurus, Application Domain, Technology Topic, Popular Technical Reports.
© 2024 PatSnap. All rights reserved.Legal|Privacy policy|Modern Slavery Act Transparency Statement|Sitemap|About US| Contact US: help@patsnap.com