Federal learning model training method for large-scale industrial chain privacy calculation
A learning model and industry chain technology, applied in computing models, computing, machine learning, etc., can solve problems such as reducing the accuracy of the federated learning model and affecting the training effect of the federated learning model, so as to improve service quality, operational efficiency, and high accuracy , the effect of reducing the degree of weight dispersion
- Summary
- Abstract
- Description
- Claims
- Application Information
AI Technical Summary
Problems solved by technology
Method used
Image
Examples
Embodiment 1
[0085] In this embodiment, for the threat model, the present invention provides an industrial chain-oriented multi-party collaborative federated learning model, such as figure 2 shown. The purpose of the multi-party collaborative computing committee is to help task issuers train a federated learning model through federated learning The federated learning worker will take the locally trained model sent to the data requester. On the one hand, in order to achieve fair and efficient federated learning, the present invention provides an incentive mechanism based on workers' data sharing rate and computing resource sharing rate to distribute rewards, so as to maximize the profit of model users. Optimize the performance of federated learning under specific resource constraints. On the other hand, in order to achieve reliable and robust federated learning, during the aggregation step, the data proxy node will eliminate the model with a large difference between the class distribu...
Embodiment 2
[0087] In this embodiment, the present invention also provides a federated learning communication model and computing model, including: considering a group of devices with federated computing capabilities The computing power (that is, the CPU cycle frequency) is expressed as f n , the number of CPU cycles required to train the local model is c n ,q n Represents the sample data size, so the calculation time of one iteration of worker n is expressed by the following formula:
[0088]
[0089] in, is the effective capacitance parameter of worker n chipset. Express the transfer rate of federated learning parameters as where B is the transmission bandwidth, ρ n is the transmission power of worker n, h n is the channel gain of the point-to-point link between the federated learning worker node and the federated learning center server node, N 0 is possible noise. use ε n Indicates the quality of the local model trained by the worker, which mainly depends on the contri...
Embodiment 3
[0099] In this embodiment, in order to perform reliable federated learning tasks and promote data sharing transactions, it is necessary for task publishers to reward nodes that perform well in training tasks so that they can be more active when future tasks come. Contribute data and train models of good quality. In the model transaction of federated learning collaborative computing, two stakeholders need to be considered, namely, the profit of the task issuer (also the model user) and the utility of the federated learning workers (model provider).
[0100] For the task issuer, it needs to design a personalized contract for each data type of worker, and each task issuer will pay the contributing federated learning workers to meet their own needs. Denote the contract signed by the task issuer and worker n as {R n (q n ),q n},R n Is the reward factor for the worker, including a series of reward packages for the worker's computing resource supply rate and data supply rate. It...
PUM
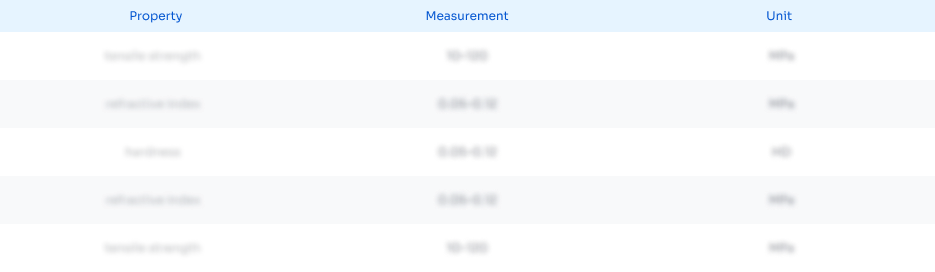
Abstract
Description
Claims
Application Information
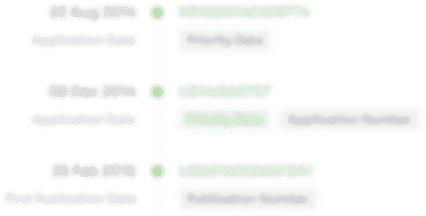
- R&D
- Intellectual Property
- Life Sciences
- Materials
- Tech Scout
- Unparalleled Data Quality
- Higher Quality Content
- 60% Fewer Hallucinations
Browse by: Latest US Patents, China's latest patents, Technical Efficacy Thesaurus, Application Domain, Technology Topic, Popular Technical Reports.
© 2025 PatSnap. All rights reserved.Legal|Privacy policy|Modern Slavery Act Transparency Statement|Sitemap|About US| Contact US: help@patsnap.com