Transient assessment evaluation feature selection method and device
A feature selection method and a feature selection technology, applied in the direction of instruments, data processing applications, resources, etc., can solve the problems that the stable samples are not considered, the unstable samples are very few, and the criteria for determining the selection threshold are not given, so as to reduce the impact , to ensure the effect of rationality
- Summary
- Abstract
- Description
- Claims
- Application Information
AI Technical Summary
Problems solved by technology
Method used
Image
Examples
Embodiment 1
[0104] Embodiment 1. Construction of sample set
[0105] This patent uses the New England 39-node system as the test system, and the system structure is as follows figure 2 As shown, it contains 10 generators, 39 nodes, 46 branches, and 19 load points. The generator model adopts a 4th-order model, and the load model is a constant impedance model.
[0106] During the operation of the power system, the power flow state of the random power grid should satisfy the power flow equation, and the equation is f(A,p,D,u,x)=0. Among them, A is an association matrix, which represents the structural variables of the network, which is determined by the topology of the power grid; p is the parameters of network components, such as transmission line parameters, transformer parameters, etc.; D is an uncontrollable variable, such as the system load in a general sense; u is a control variable , such as generator active power and machine terminal voltage, capacitive reactor switching, etc.; x ...
Embodiment 2
[0111] Embodiment 2. Construction of original input features
[0112] A key step in realizing transient stability assessment based on machine learning technology is to select reasonable state quantities as the input features of the classifier. For a specific power system, when factors such as generator distribution, generator output level, load level, load distribution, and fault conditions of the system are determined, the stability level of the system is determined. Therefore, the present invention uses the steady-state operation information before the failure as the candidate input feature set of the original feature set. When the steady-state operating variables are used as the original feature set, the evaluation speed is fast, and online evaluation does not require numerical simulation; generally, the key features identified through feature selection are the operating variables that operators should focus on monitoring. Moreover, using steady-state operating variables a...
Embodiment 3
[0115] Embodiment 3, feature selection results
[0116] The random forest algorithm needs to set two main parameters: the number of trees in the random forest and the number of preselected features in the tree nodes. The number of preselected features in the tree node takes the default value (p is the number of features in the training set), and the number of decision trees is set to 300.
[0117] The present invention compares the effect of feature selection using the WRF-RFE method formed by weighted random forest and the RF-RFE method (Random Forest-Recursive Feature Elimination) formed by ordinary random forest in the process of combining the recursive feature elimination strategy. For the class with a small number of samples, a larger weight is set, and a reasonable setting of the weight can improve the effect of feature selection. In the invention, the weight value of the stable sample and the unstable sample is set to 1:3.
[0118] According to the relationship betwe...
PUM
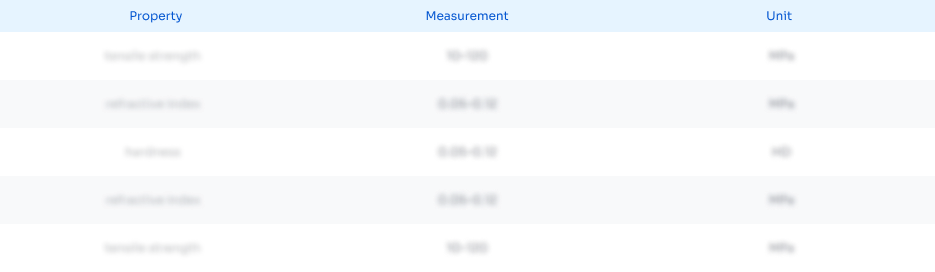
Abstract
Description
Claims
Application Information
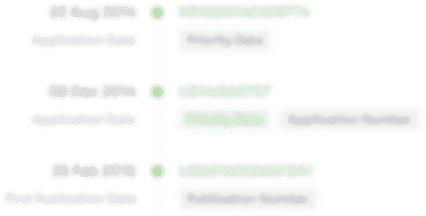
- Generate Ideas
- Intellectual Property
- Life Sciences
- Materials
- Tech Scout
- Unparalleled Data Quality
- Higher Quality Content
- 60% Fewer Hallucinations
Browse by: Latest US Patents, China's latest patents, Technical Efficacy Thesaurus, Application Domain, Technology Topic, Popular Technical Reports.
© 2025 PatSnap. All rights reserved.Legal|Privacy policy|Modern Slavery Act Transparency Statement|Sitemap|About US| Contact US: help@patsnap.com