Welding process parameter optimization method based on a BP neural network
A BP neural network and process parameter optimization technology, applied in neural learning methods, biological neural network models, design optimization/simulation, etc., can solve the problems of complex welding process debugging, low welding production efficiency and process design efficiency, etc., to achieve The effect of simplifying the process of welding parameter adjustment, flexible debugging, and simplifying the adjustment process
- Summary
- Abstract
- Description
- Claims
- Application Information
AI Technical Summary
Problems solved by technology
Method used
Image
Examples
Embodiment 1
[0020] The invention provides a process parameter optimization method based on BP neural network. The specific idea is: based on the finite element calculation method, a steel-aluminum laser welding model is established and a heat source is loaded, the heat source model is corrected through experimental temperature measurement, and then the calculated Analyze the temperature field and change law of the temperature field to obtain the training sample data of the neural network; establish a neural network model for steel-aluminum laser welding, use the training samples obtained to learn and train the neural network, and optimize the welding process parameters through the neural network; select The range of laser welding process parameters, design orthogonal experiments, obtain test results through numerical simulation, and obtain welding penetration data under different process parameters as neural network training samples; use training samples to train BP neural network to obtain...
Embodiment 2
[0022] The welding process is a complex process involving physical changes, heat transfer, mechanics, metallurgy and other aspects, including electromagnetic, heat transfer, melting and solidification, crystallization, transformation and other stages of process. It is impossible for finite element analysis software to analyze all processes All of these factors are taken into consideration, and some reasonable assumptions and simplifications should be made for the variables with weak influence when establishing the geometric model of laser welding. It avoids the problem that too much data leads to a large amount of calculation, too long time consumption or difficulty in calculation convergence. Considering that the experimental object is a steel-aluminum thin plate, the Gaussian surface heat source model is selected, and the laser power, defocus rate and welding speed are changed to obtain the penetration depth data under different process parameter combinations.
[0023] In th...
PUM
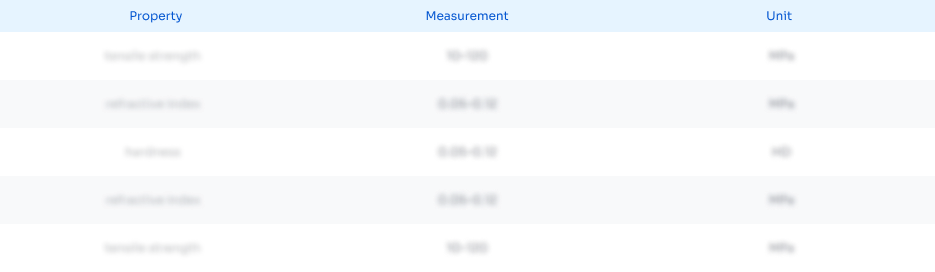
Abstract
Description
Claims
Application Information
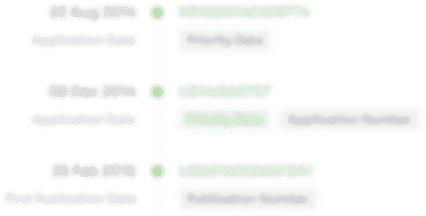
- R&D Engineer
- R&D Manager
- IP Professional
- Industry Leading Data Capabilities
- Powerful AI technology
- Patent DNA Extraction
Browse by: Latest US Patents, China's latest patents, Technical Efficacy Thesaurus, Application Domain, Technology Topic, Popular Technical Reports.
© 2024 PatSnap. All rights reserved.Legal|Privacy policy|Modern Slavery Act Transparency Statement|Sitemap|About US| Contact US: help@patsnap.com