Pulmonary nodule database and prediction model construction method and system based on column diagram
A technology of prediction model and construction method, applied in the field of data analysis, which can solve the problems of patient physical injury, complex and diverse causes of nodules, and economic burden on patients, so as to reduce overdiagnosis and treatment, rapid and reasonable clinical management, and improve treatment status. Effect
- Summary
- Abstract
- Description
- Claims
- Application Information
AI Technical Summary
Problems solved by technology
Method used
Image
Examples
Embodiment 1
[0060] The flow chart of the present invention is figure 1 As shown, by collecting 363 cases of LDCT feature information (containing 14 variable information) and serum CEA level detection results, the collected 15 variable information is analyzed for the collection of 15 variable information. And perform model build. In addition, 129 patients with 129 patients with malignant pulmonary tuberculosis were collected by LDCT information and serum CEA level results, and the robustness of model was further verified.
[0061] 1) Collect the medical record information and screening model indicators. Collect the clinical information, LDCT feature information and serum CEA level information of 363 patients with malignant pulmonary nodules of a triply hospital, and construct a good malignant lung nodubit database. The LDCT detection information includes 14 characteristic indicators, namely the number of nodules, nodules, nodules, nodules, nodule edges, empty prizes, glitch, angiogenesis, divi...
Embodiment 2
[0075] 1. Collection of serum specimens clinical information
[0076] The present invention is included in 492 cases of studies, divided into test groups and verification groups, and test groups for screening LDCT indicators with high value of good malignant tuberculosis, and construct a Logistic regression model. The verification group verifies the Logistic regression model.
[0077] The 363 patients with malignant tuberculosis included in the test group came from the lung nailing patients in Henan Province, 2016-2018. The 129 patients with lung nodules included in the verification group came from 2019-2020, diagnosis of lung nodules in the same hospital. All cases were diagnosed by histopathology, and patients with malignant lung nodules did not pass any surgical and chemotherapy. The specific medical record information is shown in Table 1.
[0078] 2. Screening and identification of LDCT indicators
[0079] A total of 14 LDCT variables (Number of nodules, nodules, nodules, nodu...
PUM
Property | Measurement | Unit |
---|---|---|
Critical value | aaaaa | aaaaa |
Abstract
Description
Claims
Application Information
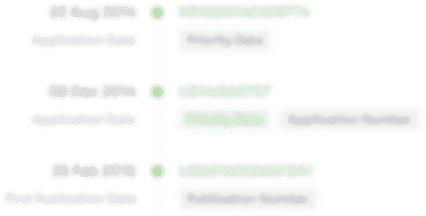
- R&D Engineer
- R&D Manager
- IP Professional
- Industry Leading Data Capabilities
- Powerful AI technology
- Patent DNA Extraction
Browse by: Latest US Patents, China's latest patents, Technical Efficacy Thesaurus, Application Domain, Technology Topic, Popular Technical Reports.
© 2024 PatSnap. All rights reserved.Legal|Privacy policy|Modern Slavery Act Transparency Statement|Sitemap|About US| Contact US: help@patsnap.com