Remaining life prediction method of lithium battery echelon utilization based on convolutional neural network
A convolutional neural network and life prediction technology, applied in neural learning methods, biological neural network models, neural architectures, etc., can solve problems such as inaccurate prediction of remaining service life, reduce energy consumption, improve economy, and save The effect of labor costs
- Summary
- Abstract
- Description
- Claims
- Application Information
AI Technical Summary
Problems solved by technology
Method used
Image
Examples
no. 1 example
[0043] Please refer to figure 1 , figure 2 , image 3 , Figure 4 and Figure 5 ,in, figure 1 It is a schematic structural diagram of the first embodiment of the method for predicting the remaining life of a lithium battery based on a convolutional neural network provided by the present invention; figure 2 for figure 1 The schematic diagram of the first operation flow shown; image 3 for figure 1 The schematic diagram of the first operation flow shown; Figure 4 for figure 1 The schematic diagram of the structure of the cell electrode localization convolutional neural network shown; Figure 5 for figure 1 Shown is a schematic diagram of the remaining service life prediction convolutional neural network structure; the remaining life prediction method of lithium battery echelon utilization based on convolutional neural network includes the following steps:
[0044] S1: Use the constant current voltage test method to obtain the battery capacity value and battery inte...
no. 2 example
[0114] Please refer to Image 6 , Figure 7 , Figure 8 and Figure 9 , based on the convolutional neural network-based remaining life prediction method for lithium batteries provided by the first embodiment of the present application, the second embodiment of the present application proposes another convolutional neural network-based remaining life prediction method for lithium batteries. The second embodiment is only a preferred mode of the first embodiment, and the implementation of the second embodiment will not affect the independent implementation of the first embodiment.
[0115] Specifically, the difference between the convolutional neural network-based remaining life prediction method of lithium battery echelon utilization provided by the second embodiment of the present application is that the convolutional neural network-based lithium battery echelon utilization remaining life prediction method, the scanning The module also includes a scanning table 1, a surface ...
PUM
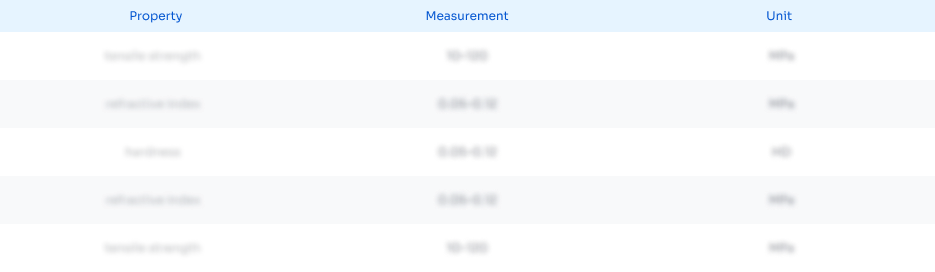
Abstract
Description
Claims
Application Information
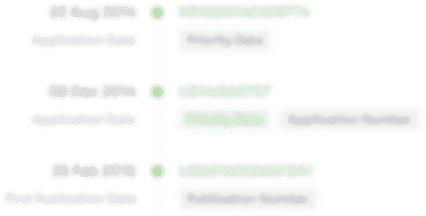
- R&D Engineer
- R&D Manager
- IP Professional
- Industry Leading Data Capabilities
- Powerful AI technology
- Patent DNA Extraction
Browse by: Latest US Patents, China's latest patents, Technical Efficacy Thesaurus, Application Domain, Technology Topic, Popular Technical Reports.
© 2024 PatSnap. All rights reserved.Legal|Privacy policy|Modern Slavery Act Transparency Statement|Sitemap|About US| Contact US: help@patsnap.com