Convolutional neural network-based low-dosage CT image noise inhibition method
A convolutional neural network and CT image technology, applied in machine learning and pattern recognition, low-dose CT image noise suppression based on convolutional neural network, to avoid cumbersome processes and preserve image details
- Summary
- Abstract
- Description
- Claims
- Application Information
AI Technical Summary
Problems solved by technology
Method used
Image
Examples
Embodiment 1
[0074] In the training process of the convolutional neural network, different purposes can be achieved by changing the preprocessing process of the data input to the network: (1) by training the convolutional neural network to predict the noise image corresponding to the low-dose CT image, after processing A denoised image can be obtained. (2) Also according to the purpose and principle of denoising low-dose CT images, the same neural network can be used to directly predict high-dose CT images corresponding to low-dose CT images through different training methods. Low-dose CT denoising is basically the same, but the detail process is slightly different. The following will describe the process of realizing the two goals.
[0075] Objective 1: Input low-dose CT images to suppress artifacts and noise in low-dose CT images, and suppress or remove noise and artifacts in low-dose CT images through the predictive ability of convolutional neural networks
[0076] The present inventi...
Embodiment 2
[0118] In the above experiments, the convolutional neural network used is a plain network formed by stacking volume-based layers. In the specific implementation, the application method of the present invention can be extended by introducing connection shortcuts between the volume-based layers in the plain network. To further improve the denoising effect, the method of introducing connection shortcuts in this experiment is relatively flexible. Only two examples are given, but the network architecture involving such connection shortcuts belongs to the protection scope of this patent.
[0119] 1. Residual network connection (multi-scale cascade) method:
[0120] In the "flat" network (and the network introduced above), when the number of network layers increases to a certain extent, the network's
[0121] The output of the layer-number convolutional neural network not only did not improve, but showed significant degradation. In order for the network to reach a deeper level witho...
Embodiment 3
[0133] The above experiments dealt with two-dimensional CT images, which can be extended to the processing of three-dimensional low-dose CT images.
[0134] It is also feasible to use convolutional neural networks to denoise 3D images or predict iteratively reconstructed high-dose CT images at the 3D level. The principle is the same as operating on a two-dimensional plane. It uses the 3D convolution kernel to extract the features of the input 3D CT image data. These convolution kernels can be operated on different levels, and more spatial details of the image are retained. A 3D convolution is constructed based on the 3D convolution feature kernel. product neural network. Compared with the two-dimensional convolutional neural network, this network architecture can generate multi-channel information from successive layers, and finally combine the information of all channels to form the final feature description.
[0135] The basic process of generating high-dose iteratively re...
PUM
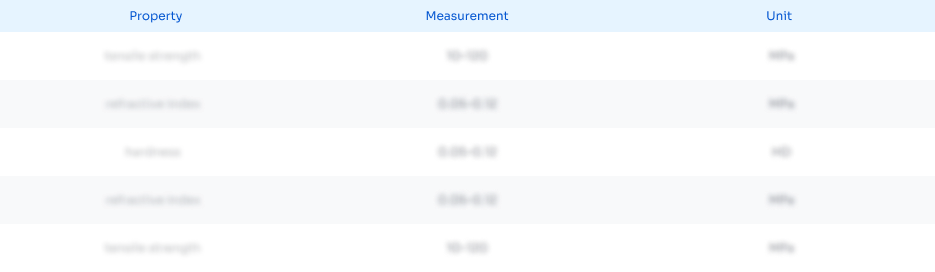
Abstract
Description
Claims
Application Information
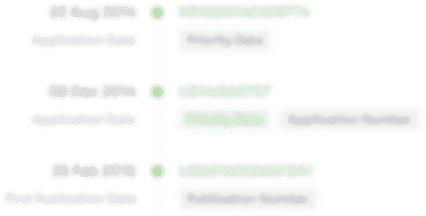
- R&D
- Intellectual Property
- Life Sciences
- Materials
- Tech Scout
- Unparalleled Data Quality
- Higher Quality Content
- 60% Fewer Hallucinations
Browse by: Latest US Patents, China's latest patents, Technical Efficacy Thesaurus, Application Domain, Technology Topic, Popular Technical Reports.
© 2025 PatSnap. All rights reserved.Legal|Privacy policy|Modern Slavery Act Transparency Statement|Sitemap|About US| Contact US: help@patsnap.com